
An estimated regression equation may be used for a wide variety of business applications, such as:
- Measuring the impact on a corporation's profits of an increase in profits
- Understanding how sensitive a corporation's sales are to changes in advertising expenditures
- Seeing how a stock price is affected by changes in interest rates
What is regression analysis and why should I use it?
- Regression analysis allows you to understand the strength of relationships between variables. ...
- Regression analysis tells you what predictors in a model are statistically significant and which are not. ...
- Regression analysis can give a confidence interval for each regression coefficient that it estimates. ...
- and much more…
When should I use regression analysis?
Use regression analysis to describe the relationships between a set of independent variables and the dependent variable. Regression analysis produces a regression equation where the coefficients represent the relationship between each independent variable and the dependent variable. You can also use the equation to make predictions. As a statistician, I should probably tell you that I love all ...
How to perform a simple regression analysis?
How to Perform a Simple Regression Analysis. The most common way people perform a simple regression analysis is by using statistical programs to enable fast analysis of the data. Performing the simple linear regression in R. R is a statistical program that is used in carrying out a simple linear regression analysis. It is widely used, powerful ...
What are the advantages and disadvantages of regression analysis?
Regression testing in agile helps in identifying the problematic areas at an early stage so that the developers can immediately replace that section with proper code, It also advantages and disadvantages of regression analysis helps achieve better software reliability. As regression testing executes the same steps repeatedly and allows the team with shorter sprints to deliver better quality products to the customer.
What is linear regression used for?
Simple linear regression is commonly used in forecasting and financial analysis —for a company to tell how a change in the GDP could affect sales, for example.
What software can do regression?
Popular business software such as Microsoft Excel can do all the regression calculations and outputs for you, but it is still important to learn the underlying mechanics.
How to find correlation between GDP and sales?
Both tend to go up together. Using Excel, all you have to do is click the Tools drop-down menu, select Data Analysis and from there choose Regression . The popup box is easy to fill in from there; your Input Y Range is your "Sales" column and your Input X Range is the change in GDP column; choose the output range for where you want the data to show up on your spreadsheet and press OK. You should see something similar to what is given in the table below:
What is the relationship between two variables in a regression model?
At the heart of a regression model is the relationship between two different variables, called the dependent and independent variables . For instance, suppose you want to forecast sales for your company and you've concluded that your company's sales go up and down depending on changes in GDP.
What does a correlation of +1 mean?
A correlation of +1 can be interpreted to suggest that both variables move perfectly positively with each other and a -1 implies they are perfectly negatively correlated. In our previous example, if the correlation is +1 and the GDP increases by 1%, then sales would increase by 1%. If the correlation is -1, a 1% increase in GDP would result in a 1% decrease in sales—the exact opposite.
Why do we need to standardize covariance?
We need to standardize the covariance in order to allow us to better interpret and use it in forecasting, and the result is the correlation calculation . The correlation calculation simply takes the covariance and divides it by the product of the standard deviation of the two variables. This will bind the correlation between a value of -1 and +1.
What is the formula for calculating the relationship between two variables?
The formula to calculate the relationship between two variables is called covariance. This calculation shows you the direction of the relationship. If one variable increases and the other variable tends to also increase, the covariance would be positive.
How does regression analysis help a business?
Regression analysis can help businesses plot data points like sales numbers against new business launches, like new products, new POS systems, new website launch, etc. Regression analysis can help a business see – over both the short and long term – the effect that these moves had on the bottom line and also help businesses work backwards to see if changes in their business model influenced other parts of your business.
Why is regression analysis important?
It helps businesses understand the data points they have and use them – specifically the relationships between data points – to make better decisions, including anything from predicting sales to understanding inventory levels and supply and demand. Of all the business analysis techniques, regression analysis is often referred to as one ...
Who handles the regression data analysis?
Regression data analysis can create huge amounts of value for a company, but who’s in charge of this kind of analysis? And who benefits the most? Business analysts and data professionals are often the ones that can pull the relevant data and create reports for department heads, management teams, sales units, board members, or anyone looking for significant data to guide or support decisions.
Why is it important to optimize processes?
Optimizing processes: Having more data, and an understanding of that data, can help to maximize efficiency and refine processes so that businesses can get the most out of them. Processes that are optimized by statistical data can help businesses work smarter.
How to use regression analysis in business?
The uses of regression analysis in business can influence every aspect of your company, from efficient use of resources to planning marketing efforts for maximum impact. The key is to collect accurate data for unbiased results and correctly choose the independent and dependent variables for each regression analysis. From there, you don't need to worry too much about remembering your high school algebra classes. You can use spreadsheet software like Microsoft Excel or Google Sheets to run a regression analysis and then interpret the results yourself.
Why is regression important in business?
The application of regression analysis in business helps show a correlation (or lack thereof) between two variables. Using basic algebra, you can determine whether one set of data depends on another set of data in a cause-and-effect relationship. You might already suspect that your sales figures depend on the time of day, for example, but a regression analysis provides hard evidence to prove or disprove your hypothesis.
How many data sets are needed for regression analysis?
Keep in mind that the more data points you can collect, the more accurate the regression analysis will be. An ideal minimum target is 100 data sets. Anything less than 25 sets is unreliable, and the more sets you have, the more accurate your analysis will be. In scenarios where the data is limited to begin with, just work with what you can get and take the results with a grain of salt.
Why is it important to collect accurate data?
First, you'll want to make sure that the data you collect is accurate. Accurate data is crucial for reducing the margin of error. You don't want to get a false positive and completely revamp a process or campaign for little to no return. In general, automated computer systems will give you better results than handwritten data collection.
What is the R-squared statistic?
R-squared, also called the coefficient of determination, is one such statistic, and it shows how closely the data points match the line of best fit on a scale of 0 to 1. In other words, if you were to plot the data points on a graph and draw a straight line through them, would the line pass through each of the data points? If so, R-squared would have a value of 1, but that would be in a perfect scenario.
Is the dependent variable always a guess?
Determining the dependent variable for a regression analysis is always just a guess in the beginning. The dependent variable may not depend on the independent variable whatsoever. That's the point of the regression analysis: Does this variable depend on this other variable?
Is a higher R squared better?
In general, a higher R-squared is desirable. However, lower R-squared values don't automatically mean there's no correlation between data sets, especially when the dependent variable is a human behavior. After all, human behavior is inherently unpredictable.
What is the purpose of regression analysis?
A regression analysis is a tool that can be used to separate variables that matter from variables that do not. The ultimate goal of a regression analysis is to understand whether A is related to B. When there is a link between A and B, the relationship is called a correlation. If we can say that the nature of the link is such that A actually affects (causes, eliminates, or amplifies) B, a relationship of cause is established.
Why is regression conducted?
In technical terms, a regression is conducted for the purpose of ''thinning the herd'' of variables in order to find the ones that actually matter. If a regression has been constructed and analyzed correctly, it will establish the presence or absence of a relationship, but it is still not necessarily a cause relationship between variables.
How Do We Know That This Data Actually Means Anything?
Plotting data points in a regression is how statisticians determine whether or not a relationship exists. Graphing the results of a regression is one of the most effective ways of presenting data gathered from a regression analysis. The graph is created by plotting a dependent variable on one axis and an independent variable on the other axis. The data points are placed on the graph and a pattern (or lack thereof) emerges. Let's look at an example to help better explain this process.
Why is graphing data important?
Graphing the data is important because the trend line on the graph is essentially the answer to the question. When a regression is plotted and the result shows no pattern (Figure 1), it is unlikely that there is any relationship between the variables at all.
What is a correlation in sales pitch?
This is called a correlation. A correlation is an observation that two things seem to happen with frequency, but it does not speak to whether the variable is actually causing the occurrence. To better illustrate the point, take a look at some exaggerated cases of correlation without a true relationship:
What is it called when data points fall outside the normal distribution?
Data points falling far outside the normal distribution are called outliers and they must be removed before performing the regression so that they do not skew the results of the regression. To unlock this lesson you must be a Study.com Member. Create your account.
What is the highest order of statistical relationships?
The highest order of statistical relationships, the causal relationship, is a plot with a very symmetrical appearance . The graph in figure 4 could be in response to a research question like, ''Do sales go up if we offer free coffee in the store?''
What is regression analysis?
Regression analysis is a way of mathematically sorting out which of those variables does indeed have an impact. It answers the questions: Which factors matter most? Which can we ignore? How do those factors interact with each other? And, perhaps most importantly, how certain are we about all of these factors?
What is the main factor in regression analysis?
In regression analysis, those factors are called variables. You have your dependent variable — the main factor that you’re trying to understand or predict. In Redman’s example above, the dependent variable is monthly sales. And then you have your independent variables — the factors you suspect have an impact on your dependent variable.
How do companies use it?
Regression analysis is the “go-to method in analytics,” says Redman. And smart companies use it to make decisions about all sorts of business issues. “As managers, we want to figure out how we can impact sales or employee retention or recruiting the best people. It helps us figure out what we can do.”
Why does a regression line always have an error term?
A regression line always has an error term because, in real life, independent variables are never perfect predictors of the dependent variables. Rather the line is an estimate based on the available data. So the error term tells you how certain you can be about the formula. The larger it is, the less certain the regression line.
What is the red line in statistics?
In other words, explains Redman, “The red line is the best explanation of the relationship between the independent variable and dependent variable.”
What is the point of analysis?
The point of the analysis is to quantify the certainty that something will happen. “It’s not telling you how rain will influence your sales, but it’s telling you the probability that rain may influence your sales.”. The last mistake that Redman warns against is letting data replace your intuition.
What to keep in mind when doing regression analysis?
First, don’t tell your data analyst to go out and figure out what is affecting sales. “The way most analyses go haywire is the manager hasn’t narrowed the focus on what he or she is looking for,” says Redman.
What is regression analysis?
Regression analysis is the mathematically measured correlation of a link between two variables: the independent variable X and the dependent variable Y. Regression analysis evaluates how strongly related the two elements are in order to help you make stronger business plans, decisions and forecasts. For example, it can help you better understand the relationship between variables that affect your sales or budgeting goals.
What to do if regression analysis doesn't seem right?
If the data you receive from your regression analysis doesn't seem right or if the study's error terms are off, ask a more experienced colleague for their opinion and learn when to look at both the analysis results and the human aspects that affect an outcome.
What is the letter A in regression?
In a simple regression analysis formula, the letter A refers to the dependent variable and the value of Y when x = 0. B refers to the slope of the correlation, and U is the residual error. The formula to predict how data may look in the future is:
How does regression analysis work?
In order to conduct a regression analysis, you’ll need to define a dependent variable that you hypothesize is being influenced by one or several independent variables.
What is the formula for regression line?
The formula for a regression line might look something like Y = 100 + 7X + error term.
Why is regression line an error term?
Regression lines always consider an error term because in reality, independent variables are never precisely perfect predictors of dependent variables. This makes sense while looking at the impact of ticket prices on event satisfaction — there are clearly other variables that are contributing to event satisfaction outside of price.
Why do we distribute follow up surveys?
In order to understand the value being delivered at these training events , we distribute follow-up surveys to attendees with the goals of learning what they enjoyed, what they didn’t, and what we can improve on for future sessions.
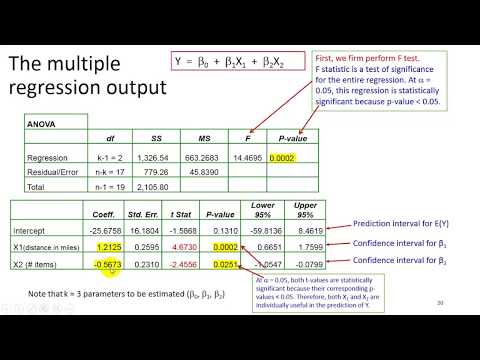
Variables
Covariance
- Cov(x,y)=∑(xn−xu)(yn−yu)N\begin{aligned} &Cov(x,y) = \sum \frac { ( x_n - x_u )( y_n - y_u) }{ N } \\ \end{aligned}Cov(x,y)=∑N(xn−xu)(yn−yu) The formula to calculate the relationship between two variables is called covariance. This calculation shows you the direction of the relationship. If one variable increases and the other variable tends to also increase, the covariance would be p…
Correlation Coefficient
- Correlation=ρxy=Covxysxsy\begin{aligned} &Correlation = \rho_{xy} = \frac { Cov_{xy} }{ s_x s_y } \\ \end{aligned}Correlation=ρxy=sxsyCovxy We need to standardize the covariance in order to allow us to better interpret and use it in forecasting, and the result is the correlation calculation. The correlation calculation simply takes the covariance and divides it by the product …
Regression Equation
- Now that we know how the relative relationship between the two variables is calculated, we can develop a regression equation to forecast or predict the variable we desire. Below is the formula for a simple linear regression. The "y" is the value we are trying to forecast, the "b" is the slope of the regression line, the "x" is the value of our independent value, and the "a" represents the y-int…
Regressions in Excel
- Now that you understand some of the background that goes into a regression analysis, let's do a simple example using Excel's regression tools. We'll build on the previous example of trying to forecast next year's sales based on changes in GDP. The next table lists some artificial data points, but these numbers can be easily accessible in real life. Just eyeballing the table, you ca…
Interpretation
- The major outputs you need to be concerned about for simple linear regression are the R-squared, the intercept (constant) and the GDP's beta (b) coefficient. The R-squared number in this example is 68.7%. This shows how well our model predicts or forecasts the future sales, suggesting that the explanatory variables in the model predicted 68.7% of the variation in the de…
The Bottom Line
- So how would you use this simple model in your business? Well if your research leads you to believe that the next GDP change will be a certain percentage, you can plug that percentage into the model and generate a sales forecast. This can help you develop a more objective plan and budget for the upcoming year. Of course, this is just a simple regression and there are models th…