
The p value determines statistical significance. An extremely low p value indicates high statistical significance, while a high p value means low or no statistical significance. Example: Hypothesis testing To test your hypothesis, you first collect data from two groups. The experimental group actively smiles, while the control group does not.
How do you test for statistical significance in research?
How do you test for statistical significance? In quantitative research, data are analyzed through null hypothesis significance testing, or hypothesis testing. This is a formal procedure for assessing whether a relationship between variables or a difference between groups is statistically significant.
How do you determine if a variable is statistically significant?
determine whether a predictor variable has a statistically significant relationship with an outcome variable. estimate the difference between two or more groups. Statistical tests assume a null hypothesis of no relationship or no difference between groups.
How do you find the difference between three groups in statistics?
If you are using categorical data you can use the Kruskal-Wallis test (the non-parametric equivalent of the one-way ANOVA) to determine group differences. If the test shows there are differences between the 3 groups. You can use the Mann-Whitney test to do pairwise comparisons as a post hoc or follow up analysis.
How do you determine if a relationship is statistically significant?
If the value of the test statistic is more extreme than the statistic calculated from the null hypothesis, then you can infer a statistically significant relationship between the predictor and outcome variables.
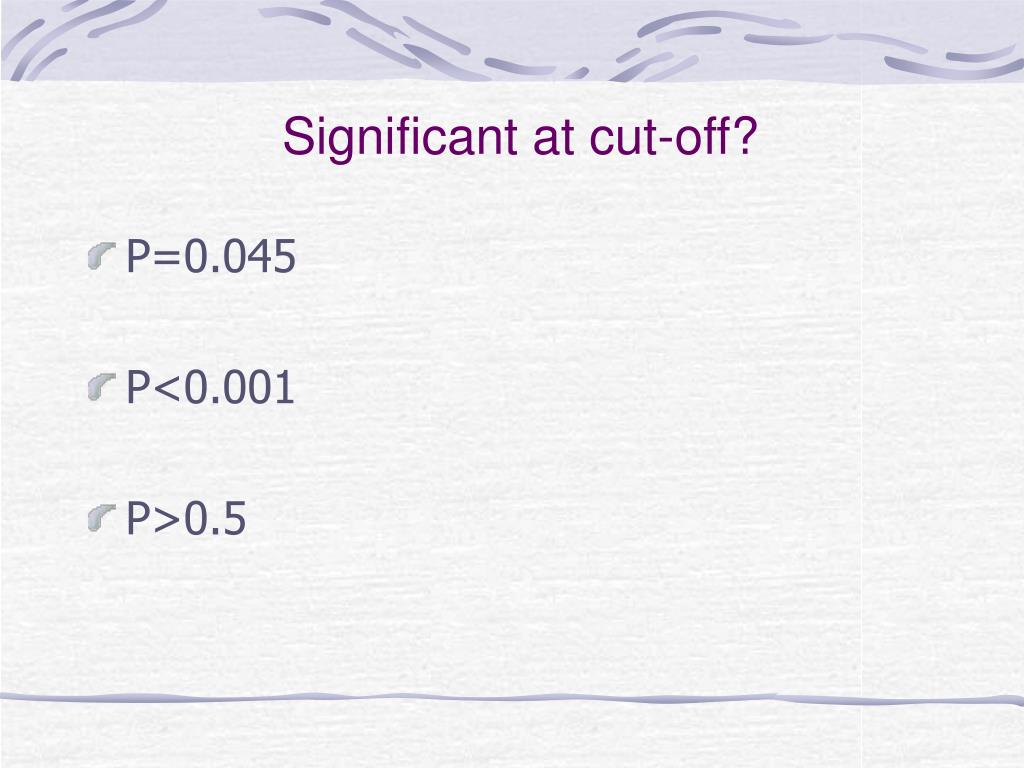
How do you test for statistical significance?
This is a formal procedure for assessing whether a relationship between variables or a difference between groups is statistically significant .
When reporting statistical significance, do you include descriptive statistics?
When reporting statistical significance, include relevant descriptive statistics about your data (e.g. means and standard deviations) as well as the test statistic and p value.
Why is the significance level higher?
This makes the study less rigorous and increases the probability of finding a statistically significant result.
What does the p value tell you about a statistically significant finding?
In other words, a statistically significant result has a very low chance of occurring if there were no true effect in a research study . The p value, or probability value, tells you the statistical significance of a finding.
How are P-values calculated?
P -values are calculated from the null distribution of the test statistic. They tell you how often a test statistic is expected to occur under the null hypothesis of the statistical test, based on where it falls in the null distribution.
Why is statistical significance misleading?
On its own, statistical significance may also be misleading because it’s affected by sample size. In extremely large samples, you’re more likely to obtain statistically significant results, even if the effect is actually small or negligible in the real world. This means that small effects are often exaggerated if they meet the significance threshold, while interesting results are ignored when they fall short of meeting the threshold.
When is the p value compared to the significance level?
In a hypothesis test, the p value is compared to the significance level to decide whether to reject the null hypothesis.
Step 1
Substitute the figures from the above example in the formula of comparative error:
Step 2
Calculate the absolute difference (d) between the two percentages of response r 1, r 2:
Step 3
Test the significance by checking whether the difference calculated above (d) is greater than the comparative error this way:
What is the significance level of a p-value?
The term "statistical significance" or "significance level" is often used in conjunction to the p-value, either to say that a result is "statistically significant", which has a specific meaning in statistical inference ( see interpretation below ), or to refer to the percentage representation the level of significance: (1 - p value), e.g. a p-value of 0.05 is equivalent to significance level of 95% (1 - 0.05 * 100). A significance level can also be expressed as a T-score or Z-score, e.g. a result would be considered significant only if the Z-score is in the critical region above 1.96 (equivalent to a p-value of 0.025).
What do you need to know when entering proportions data?
If entering proportions data, you need to know the sample sizes of the two groups as well as the number or rate of events. You can enter that as a proportion (e.g. 0.10), percentage (e.g. 10%) or just the raw number of events (e.g. 50).
What is a p-value?
The p-value is a heavily used test statistic that quantifies the uncertainty of a given measurement, usually as a part of an experiment, medical trial, as well as in observational studies. By definition, it is inseparable from inference through a Null-Hypothesis Statistical Test (NHST). In it we pose a null hypothesis reflecting the currently established theory or a model of the world we don't want to dismiss without solid evidence (the tested hypothesis), and an alternative hypothesis: an alternative model of the world. For example, the statistical null hypothesis could be that exposure to ultraviolet light for prolonged periods of time has positive or neutral effects regarding developing skin cancer, while the alternative hypothesis can be that it has a negative effect on development of skin cancer.
What does a p-value calculator do?
The p-value calculator will output: p-value, significance level, T-score or Z-score (depending on the choice of statistical hypothesis test), degrees of freedom, and the observed difference. For means data it will also output the sample sizes, means, and pooled standard error of the mean. The p-value is for a one-sided hypothesis (one-tailed test), allowing you to infer the direction of the effect (more on one vs. two-tailed tests ). However, the probability value for the two-sided hypothesis (two-tailed p-value) is also calculated and displayed, although it should see little to no practical applications.
When comparing two independent groups and the variable of interest is the relative (a.k.a. relative change,?
When comparing two independent groups and the variable of interest is the relative (a.k.a. relative change, relative difference, percent change, percentage difference), as opposed to the absolute difference between the two means or proportions, the standard deviation of the variable is different which compels a different way of calculating p-values [5]. The need for a different statistical test is due to the fact that in calculating relative difference involves performing an additional division by a random variable: the event rate of the control during the experiment which adds more variance to the estimation and the resulting statistical significance is usually higher (the result will be less statistically significant). What this means is that p-values from a statistical hypothesis test for absolute difference in means would nominally meet the significance level, but they will be inadequate given the statistical inference for the hypothesis at hand.
What is the p-value of a one-tailed test?
Another way to think of the p-value is as a more user-friendly expression of how many standard deviations away from the normal a given observation is. For example, in a one-tailed test of significance for a normally-distributed variable like the difference of two means, a result which is 1.6448 standard deviations away (1.6448σ) results in a p-value of 0.05.
What is the formula for calculating a p-value?
When calculating a p-value using the Z-distribution the formula is Φ (Z) or Φ (-Z) for lower and upper-tailed tests, respectively. Φ is the standard normal cumulative distribution function and a Z-score is computed.
What do you need to know to determine which statistical test to use?
To determine which statistical test to use, you need to know: whether your data meets certain assumptions. the types of variables that you’re dealing with.
How does a statistical test work?
Statistical tests work by calculating a test statistic – a number that describes how much the relationship between variables in your test differs from the null hypothesis of no relationship.
What happens if the test statistic is less extreme than the one calculated from the null hypothesis?
If the value of the test statistic is less extreme than the one calculated from the null hypothesis, then you can infer no statistically significant relationship between the predictor and outcome variables.
What is statistical test?
They can be used to: determine whether a predictor variable has a statistically significant relationship with an outcome variable. estimate the difference between two or more groups. Statistical tests assume a null hypothesis of no relationship or no difference between groups.
What happens if you don't meet the assumptions of normality or homogeneity of variance?
If your data do not meet the assumptions of normality or homogeneity of variance, you may be able to perform a nonparametric statistical test, which allows you to make comparisons without any assumptions about the data distribution.
Why are non-parametric tests useful?
Non-parametric tests don’t make as many assumptions about the data , and are useful when one or more of the common statistical assumptions are violated. However, the inferences they make aren’t as strong as with parametric tests.
Which test is more rigorous, parametric or nonparametric?
Parametric tests usually have stricter requirements than nonparametric tests, and are able to make stronger inferences from the data. They can only be conducted with data that adheres to the common assumptions of statistical tests.
What test is used to determine the difference between two groups?
If you are using categorical data you can use the Kruskal-Wallis test (the non-parametric equivalent of the one-way ANOVA) to determine group differences. If the test shows there are differences between the 3 groups. You can use the Mann-Whitney test to do pairwise comparisons as a post hoc or follow up analysis.
Why do I think chi square tests between each two groups represent good chose?
I think chi-square test between each two groups represent good chose because the chi-square test of three categories give a general decision for not equality between groups and not determine the pair comparisons.
What test is used to determine if a data is normal?
You test for normality using the shapiro wilk test , if you observe insignificant p value it means the data is normally distributed then do parametric tests ( anova test mean differences between more than 2 means- this means you may generate scores first; and then multiple comparison or post hoc tests after anova test only when the mean differences are significant). For non-parametric tests use the Kruskal-Wallis equivalent of the one-way ANOVA to determine mean differences. If the test shows there are differences between the means use Mann-Whitney test to do a post hoc analysis.
What test to use for a post hoc comparison?
If the test shows there are differences between the 3 groups. You can use the Mann-Whitney test to do pairwise comparisons as a post hoc or follow up analysis. Since you're only doing a few comparisons (i.e. X vs. Y, X, vs Z, and Y vs. Z) you wouldn't have to worry about family wise error rates.
Can you do multiple comparisons with a chi square?
Looking back at your question, I see that you seem to be thinking that you cannot do multiple comparisons with a Pearson's chi square. Black boxes such as SPSS or SAS will do it for you automatically, But we should have given the simple answer that after the 2X3 test you can run a 2X2 Pearson's chi square for each comparison. Percentages usually do not fit the assumptions of the ANOVA, unless the sample size is huge even under the popular conversions. This is because the ANOVA is rather sensitive to skew, But if the sample is above, say 400 per comparison, then Pearson's chi square will possibly be hyper sensitive. So, maybe that might be a sufficient definition of "huge" enough for the ANOVA. And at some point in your choice of a sample size the central limit theorem (CLT) will protect you from all but the most extreme non normality (ie. if one of the samples is less than a few percent.) The CLT will cause the sums and means within a test to approach normality as the sample size grows very large. Therefore the distribution of the raw data does not need to pass a normality test if the sample is very large (greater than 30 per comparison and, in the ANOVA additionally that the df for error>20). I might as well point out that assuming your data is counts, you will not have mush sensitivity (power) to detect a difference of, say a few percent, unless your total sample size is greater than say 1500.
Can you do chi squared in SPSS?
With SPSS, you can run a chi-square, and test for pair-wise differences between the pair of groups. Put your 3 groups in columns, and "ride the tube: yes/no in the rows. Use the raw numbers in the 6 cells. Select the option to use Bonferroni corrections for the pairwise comparisons.
Do percentages fit the assumptions of ANOVA?
Percentages usually do not fit the assumptions of the ANOVA, unless the sample size is huge even under the popular conversions. This is because the ANOVA is rather sensitive to skew, But if the sample is above, say 400 per comparison, then Pearson's chi square will possibly be hyper sensitive.
What variables are used to determine if a person is pregnant or not?
If you have two variables in your data, Pregnant (binary variable taking values 'Yes' and 'No') and Age (continuous). If you want to test whether there is a significant difference in the mean age between those who are Pregnant and those who are not, you might consider 't.test' in R under the assumption of unequal variance.
Can you state an assumption based on a p-value?
Then you can state you assumption based on the p-value.
Is age a categorical variable?
One of my demographic variable is age. Age is measured as continues data not categorical. If I want to test differences between two groups to determine whether there is significant difference between them or not, shall I use independent t-test, or I have to covert it to categorical variable then calculate chi-square.
Should you make sure you fulfill the assumptions made in a independent t-test?
You should make sure you fulfill the assumptions made in a independent t-test (e.g. normality ). Check Field or other literature/ the internet concerning assumptions.
Can you lose power if you convert a variable to a categorical variable?
Converting your variable to a categorical variable (and using chi-square test consequently) will make you lose power, so that would not be advisory.
When you compare medians, should you stop and ask yourself?
Popular Answers (1) When you compare medians, you should stop and ask yourself if you are interested in the difference in the medians of the two groups, or in the median difference between the observations in the two groups. The median difference is an interesting measure of effect size.
What is rank sum test?
rank-sum test. are some non-parametric statistical tests that can be applied in testing the significance of difference between the two medians of two groups. It is yet to develop the parametric test statistic for this purpose. However, if the parent population from which the sample is drawn is normal then.
What is the name of the procedure used in Stata?
Stata has a nice procedure for quantile regression, that can be used for medians and allows for inclusion of covariates in the models, including a simple contrast between two groups. The name of the procedure is "qreg".
What is the valid test for medians?
Valid tests of medians are: Mood's test and permutation test of differences in medians. But the permutation method is correct if and only if the scale parameters are equal, so the principle of data exchangeability is held. Otherwise, observations cannot be swapped between the groups and the test doesn't make any sense.
Does Man-Whitney test for equality of medians?
Man-W hitney (-Wilcoxon) and Kruskal-Wallis do not test for equality of medians in general. Please refer to the null hypothesis. In very specific cases, involving equal scale parameters and symmetry of distributions (so, when the parametric test would work well, instead) it may (and happens to) work this way.
Can you bootstrap a 95% confidence interval?
Yes, Bootstrapping method is an ideal way to do this. Basically for each median, you can re-sample your sample with replacement for a large number of times (e.g. 1000 times with a sample n=100). In doing so, you will get a normal distribution of the median you have and have a standard error. Thus, you will be able to get 95% CI. Finally, you can check if the two 95% CIs overlap. If they do, it implies the two median are not statistically significant.
Can observations be swapped between groups?
Otherwise, observations cannot be swapped between the groups and the test doesn't make any sense. Of course, if one doesn't need a p-value, and is fine with a confidence interval (which includes the statistical significance in it), bootstrapped CI of differences in medians can be calculated.
Why do we have to parse the entire statistical protocol in an well structured decision tree /algorithmic?
we have to parse the entire statistical protocol in an well structured - decision tree /algorithmic manner, in order to avoid some mistakes.
What is the most common method of inference?
4. Statistical hypothesis testing - last but not least, probably the most common way to do statistical inference is to use a statistical hypothesis testing. This is a method of making statistical decisions using experimental data and these decisions are almost always made using so-called “null-hypothesis” tests.
What is the null hypothesis in a second parameter?
That is to say, if in an estimation of a second parameter, we observed a value less than x or greater than y, we would reject the null hypothesis. In this case, the null hypothesis is: “the true value of this parameter equals P”, at the α level of significance; and conversely, if the estimate of the second parameter lay within the interval [x, y], we would be unable to reject the null hypothesis that the parameter equaled P.
What is the null hypothesis?
The null hypothesis (H0) formally describes some aspect of the statistical behavior of a set of data and this description is treated as valid unless the actual behavior of the data contradicts this assumption.
How to interpret a one tail P-value?
To interpret a one-tail P-value, we must predict which group will have the larger mean before collecting any data. The one-tail P-value answers this question: Assuming the null hypothesis is true, what is the chance that randomly selected samples would have means as far apart (or further) as observed in this experiment with the specified group having the larger mean?
What are some aspects related to the data we collected during the research/experiment?
what types of data we have - nominal, ordinal, interval or ratio , how the data are organized, how many study groups (usually experimental and control at least) we have, are the groups paired or unpaired, and are the sample (s) extracted from a normally distributed/Gaussian population);
What is the act or process of deriving a logical consequence conclusion from premises?
Answer 1: Inference is the act or process of deriving a logical consequence conclusion from premises. Statistical inference or statistical induction comprises the use of statistics and (random) sampling to make inferences concerning some unknown aspect of a statistical population (1,2).
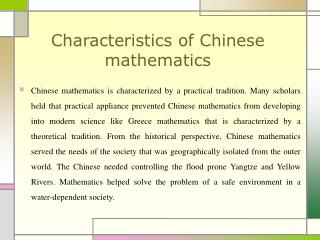
How Do You Test For Statistical significance?
- In quantitative research, data are analyzed through null hypothesis significance testing, or hypothesis testing. This is a formal procedure for assessing whether a relationship between variables or a difference between groups is statistically significant.
What Is A Significance level?
- The significance level, or alpha (α), is a value that the researcher sets in advance as the threshold for statistical significance. It is the maximum risk of making a false positive conclusion (Type I error) that you are willing to accept. In a hypothesis test, the pvalue is compared to the significance level to decide whether to reject the null hypothesis. 1. If thep value is higher than t…
Problems with Relying on Statistical Significance
- There are various critiques of the concept of statistical significance and how it is used in research. Researchers classify results as statistically significant or non-significant using a conventional threshold that lacks any theoretical or practical basis. This means that even a tiny 0.001 decrease in a pvalue can convert a research finding from statistically non-significant to si…
Other Types of Significance in Research
- Aside from statistical significance, clinical significance and practical significance are also important research outcomes. Practical significance shows you whether the research outcome is important enough to be meaningful in the real world. It’s indicated by the effect sizeof the study. Clinical significanceis relevant for intervention and treatment studies. A treatment is considered …