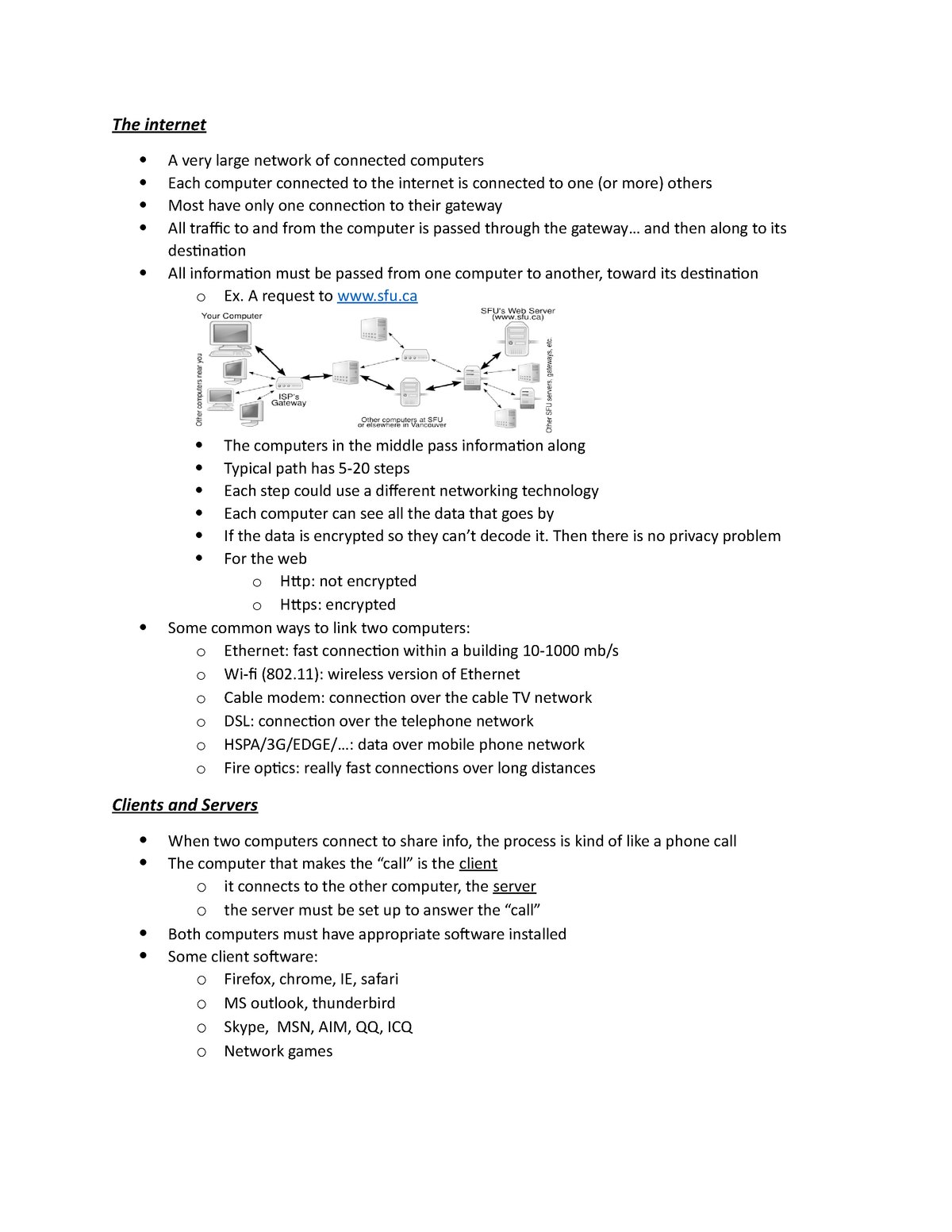
How do you find a type 1 error?
The probability of making a type I error is represented by your alpha level (α), which is the p-value below which you reject the null hypothesis. A p-value of 0.05 indicates that you are willing to accept a 5% chance that you are wrong when you reject the null hypothesis.
How do you determine Type 1 and Type 2 errors?
A type I error (false-positive) occurs if an investigator rejects a null hypothesis that is actually true in the population; a type II error (false-negative) occurs if the investigator fails to reject a null hypothesis that is actually false in the population.
What is a Type 1 error in statistics example?
In statistical hypothesis testing, a type I error is the mistaken rejection of an actually true null hypothesis (also known as a "false positive" finding or conclusion; example: "an innocent person is convicted"), while a type II error is the failure to reject a null hypothesis that is actually false (also known as a " ...
What is a Type 1 error represented by?
A Type I error is often represented by the Greek letter alpha (α) and a Type II error by the Greek letter beta (β ). In choosing a level of probability for a test, you are actually deciding how much you want to risk committing a Type I error—rejecting the null hypothesis when it is, in fact, true.
What is Type 1 Type 2 Type 3 error?
Type I error: "rejecting the null hypothesis when it is true". Type II error: "failing to reject the null hypothesis when it is false". Type III error: "correctly rejecting the null hypothesis for the wrong reason".
How do you determine Type 2 error?
How to Calculate the Probability of a Type II Error for a Specific Significance Test when Given the Power. Step 1: Identify the given power value. Step 2: Use the formula 1 - Power = P(Type II Error) to calculate the probability of the Type II Error.
What is a Type 1 error in statistics quizlet?
Type 1 error (false positive) When we accept the difference/relationship is a real one and we are wrong. A null hypothesis is rejected when it is actually true. Type 1 example. We reject a null hypothesis, stating a drug has an effect on a disease, when in reality it has no effect at all, and it is a false claim.
What is Type II error explain with example?
A type II error produces a false negative, also known as an error of omission. For example, a test for a disease may report a negative result when the patient is infected. This is a type II error because we accept the conclusion of the test as negative, even though it is incorrect.
What does a type 1 error of .05 mean?
a 5% chance05 means that there is a 5% chance of making a type I error. A type I error, exists if the Null Hypothesis is incorrectly rejected.
What are Type I and Type II errors?
In statistics, a Type I error means rejecting the null hypothesis when it’s actually true, while a Type II error means failing to reject the nu...
How do you reduce the risk of making a Type I error?
The risk of making a Type I error is the significance level (or alpha) that you choose. That’s a value that you set at the beginning of your st...
How do you reduce the risk of making a Type II error?
The risk of making a Type II error is inversely related to the statistical power of a test. Power is the extent to which a test can correctly d...
What is statistical significance?
Statistical significance is a term used by researchers to state that it is unlikely their observations could have occurred under the null hypothe...
What is statistical power?
In statistics, power refers to the likelihood of a hypothesis test detecting a true effect if there is one. A statistically powerful test is more...
What is a type 1 error?
A Type I error means rejecting the null hypothesis when it’s actually true. It means concluding that results are statistically significant when, in reality, they came about purely by chance or because of unrelated factors.
What is the difference between a type 1 error and a type 2 error?
In statistics, a Type I error is a false positive conclusion, while a Type II error is a false negative conclusion. Making a statistical decision always involves uncertainties, so the risks of making these errors are unavoidable in hypothesis testing. The probability of making a Type I error is the significance level, or alpha (α), ...
What happens if you don't reject a null hypothesis?
If your findings do not show statistical significance, they have a high chance of occurring if the null hypothesis is true. Therefore, you fail to reject your null hypothesis. But sometimes, this may be a Type II error. Example: Type I and Type II errors.
What is hypothesis error?
Using hypothesis testing, you can make decisions about whether your data support or refute your research predictions. Hypothesis testing starts with the assumption of no difference between groups or no relationship between variables in the population—this is the null hypothesis.
How do Type I and Type II error rates affect each other?
The Type I and Type II error rates influence each other. That’s because the significance level (the Type I error rate) affects statistical power , which is inversely related to the Type II error rate.
What is the risk of committing this error?
The risk of committing this error is the significance level (alpha or α) you choose. That’s a value that you set at the beginning of your study to assess the statistical probability of obtaining your results ( p value ).
What is the probability of making a type 1 error?
The probability of making a Type I error is the significance level, or alpha (α), while the probability of making a Type II error is beta (β). These risks can be minimized through careful planning in your study design.
What is the risk of making a type 1 error?
The risk of making a Type I error is the significance level (or alpha) that you choose . That’s a value that you set at the beginning of your study to assess the statistical probability of obtaining your results ( p value ).
How many values are within 1 standard deviation of the mean?
Around 68% of values are within 1 standard deviation of the mean.
What is standard deviation in statistics?
The standard deviation is the average amount of variability in your data set. It tells you, on average, how far each score lies from the mean.
Why is it important to assume that a parametric test is biased?
This is an important assumption of parametric statistical tests because they are sensitive to any dissimilarities. Uneven variances in samples result in biased and skewed test results.
Which method excludes the median when identifying Q1 and Q3?
The exclusive method excludes the median when identifying Q1 and Q3, while the inclusive method includes the median as a value in the data set in identifying the quartiles.
Can you use the mode to find the most frequent value?
For a nominal level, you can only use the mode to find the most frequent value. For an ordinal level or ranked data, you can also use the median to find the value in the middle of your data set. For interval or ratio levels, in addition to the mode and median, you can use the mean to find the average value.
Does interval have a true zero?
While interval and ratio data can both be categorized, ranked, and have equal spacing between adjacent values, only ratio scales have a true zero. For example, temperature in Celsius or Fahrenheit is at an interval scale because zero is not the lowest possible temperature.
What are Type 1 Errors and Significance Tests?
Significance Test: A significance test is a method that allows us to compare a data set with a hypothesis. The null hypothesis takes the form that there is no change, and the alternative hypothesis claims that there is a change.
Example 1
A college baseball team lost 80% of its games over the past three years. The baseball coach has redesigned the team and wants to know if the losing percentage has been lowered. The coach wants to conduct a 5% significance test to determine if the results are significant. What is the probability that the baseball coach will make a type I error?
Example 2
For two years, the employees in an office kept track of the number of paper-jams caused by the printing machine. A technician has redesigned the printing machine and wants to know if the paper-jam rate has changed. The technician wants to conduct a 1% significance test to determine if the results are significant.
What are Type I Errors?
Type I error is an omission that happens when a null hypothesis is reprobated during hypothesis testing. This is when it is indeed precise or positive and should not have been initially disapproved. So if a null hypothesis is erroneously rejected when it is positive, it is called a Type I error.
How to avoid type 2 errors?
To avoid type II errors, ensure the test has high statistical power. The higher the statistical power, the higher the chance of avoiding an error. Set your statistical power to be 80% and above and conduct your test.
What does it mean when a null hypothesis is rejected?
When a null hypothesis is rejected, it means a chain of circumstances has been established between the items being tested even though it is a false alarm or false positive. This could lead to an error or many errors in a test, known as a Type I error.
What are the two types of errors in a statistical test?
There are two common types of errors, type I and type II errors you'll likely encounter when testing a statistical hypothesis. The mistaken rejection of the finding or the null hypothesis is known as a type I error. In other words, type I error is the false-positive finding in hypothesis testing. Type II error on the other hand is the false-negative finding in hypothesis testing.
What does it mean when a type I error is decided at 5 percent?
In a case where Type I error is decided at 5 percent, it means in the null hypothesis (H0) , chances are there that 5 in the 100 hypotheses even if true will be rejected.
Why is the size of the sample a type I error?
The size of the sample can also lead to a Type I error because the outcome of the test will be affected. A small sample size might hide the significant level of the items being tested.
When conducting hypothesis testing, a null hypothesis is determined before carrying out the actual test?
When conducting hypothesis testing, a null hypothesis is determined before carrying out the actual test. The null hypothesis may presume that there is no chain of circumstances between the items being tested which may cause an outcome for the test.
What is a type 1 error?
A Type I (read “Type one”) error is when the person is truly innocent but the jury finds them guilty. A Type II (read “Type two”) error is when a person is truly guilty but the jury finds him/her innocent.
What is the difference between type I and type II error?
Another way to look at Type I vs. Type II errors is that a Type I error is the probability of overreacting and a Type II error is the probability of under reacting.
What is the Greek letter for a null hypothesis?
The Greek letter µ (read “mu”) is used to describe the population average of a group of data. When the null hypothesis states µ 1 = µ 2, it is a statistical way of stating that the averages of dataset 1 and dataset 2 are the same. The alternate hypothesis, µ 1 <> µ 2, is that the averages of dataset 1 and 2 are different. When you do a formal hypothesis test, it is extremely useful to define this in plain language. For our application, dataset 1 is Roger Clemens’ ERA before the alleged use of performance-enhancing drugs and dataset 2 is his ERA after alleged use. For this specific application the hypothesis can be stated:
What is the probability of committing a Type I error?
The calculated p-value of .35153 is the probability of committing a Type I Error (chance of getting it wrong). A p-value of .35 is a high probability of making a mistake, so we can not conclude that the averages are different and would fall back to the null hypothesis that Mr. Clemens’ average ERAs before and after are the same. As an exercise, try calculating the p-values for Mr. HotandCold and Mr. Consistent; you should get .524 and .000000000004973 respectively.
What is the t statistic for the average ERA before and after?
The t statistic for the average ERA before and after is approximately .95. The last step in the process is to calculate the probability of a Type I error (chances of getting it wrong). Most statistical software and industry in general refers to this a “p-value”.
What is a t-test?
A t-Test is the hypothesis test used to compare two different averages. There are other hypothesis tests used to compare variance (F-Test), proportions (Test of Proportions), etc. In the case of the Hypothesis test the hypothesis is specifically:
How to perform a hypothesis test?
To perform a hypothesis test, we start with two mutually exclusive hypotheses. Here’s an example: when someone is accused of a crime, we put them on trial to determine their innocence or guilt. In this classic case, the two possibilities are the defendant is not guilty (innocent of the crime) or the defendant is guilty. This is classically written as…
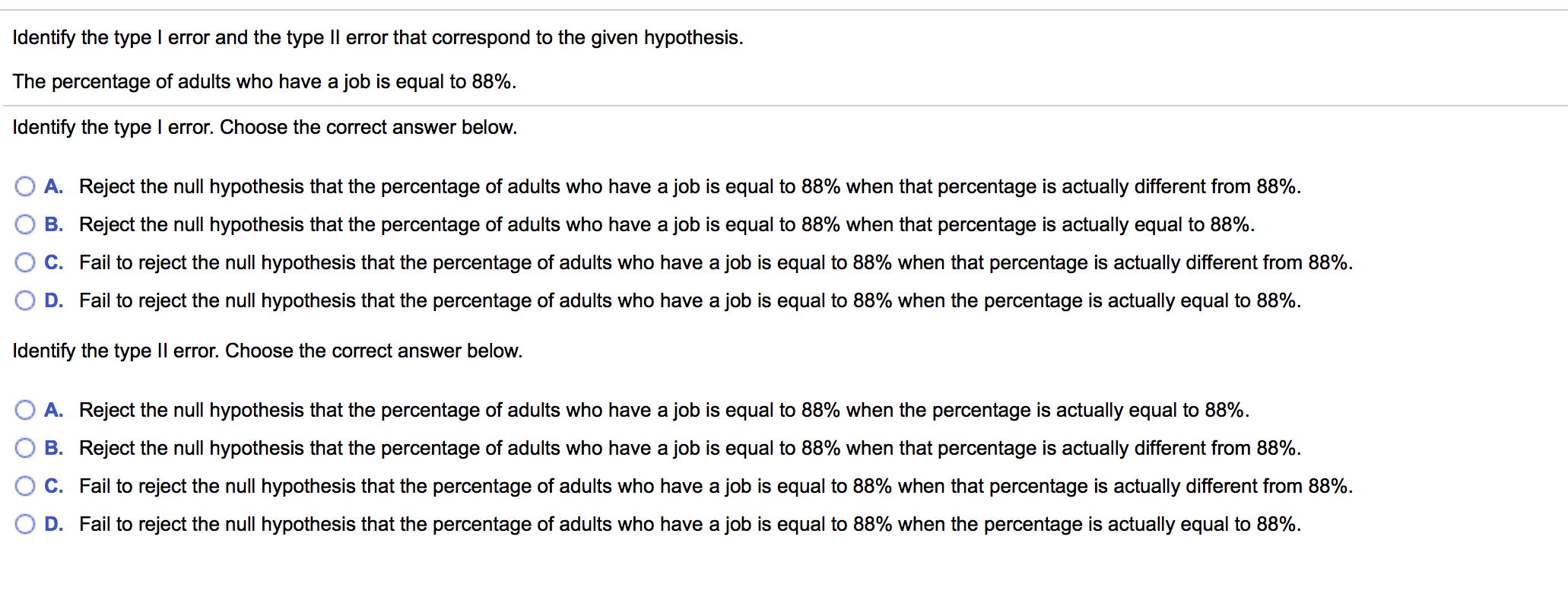