
What is the use of Hausman test in data analysis?
Hausman test is also useful for in panel data, when comparing the estimates of the fixed and random effects models. 2.4. Hausman test for differentiating between fixed effects model and random effects model
What is the best way to conduct the robust Hausman test?
The Robust Hausman Test Wooldridge (2002) explains that the easiest way to conduct the robust Hausman test is to conduct a Waldtest on the null hypothesis that H0: = 0 using the following regression:4
What is the Hausman test for endogeneity?
Hausman test for endogeneity – general case There is a group of tests named after Hausman, which are used in model selection and compare the estimators of the tested models.
Does-Hausman test tell you the right specification?
Hence, the main question is not whether -hausman. test tells you something substantive about your dataset and points you to the right specification, but whether a simple panel data regression is meaningful at all for your research purposes. As an aside, Marcos' recommendation about using CODE delimiters is highly c ommendable.

How do you choose between fixed and random effects Hausman?
1 Fixed or random. You can run a Hausman test (which tests whether the unique errors are correlated with the regressors, the null is they are not). If the p-value is significant, then you choose fixed effects (since the unique errors are correlated with the regressors).
How do you read Hausman results in eviews?
3:064:10Hausman Test in Eviews - YouTubeYouTubeStart of suggested clipEnd of suggested clipIt means that the null hypothesis is not rejected. And the statement of null hypothesis of Houseman.MoreIt means that the null hypothesis is not rejected. And the statement of null hypothesis of Houseman. Test is that the random effect regulation is appropriate.
Is Hausman test necessary?
Yes, is the sign post to know which way between fixed effects and random effects. Hausman test is used in testing for the cause- effect relationship between the dependent and independent variables in a model. The two widely used panel regression estimation techniques are fixed and random effect.
How do you test for endogeneity in panel data?
0:006:22Testing Endogeneity in Panel Data Regression using Eviews - YouTubeYouTubeStart of suggested clipEnd of suggested clipHello in this tutorial we would test image energy from the panel data integration equations usingMoreHello in this tutorial we would test image energy from the panel data integration equations using eviews let's see how to do that first of all I will open my panel data using eviews.
What is the Hausman test explain its relevance?
The Hausman test (also called the Durbin-Wu-Hausman test) is used to check whether an estimate for an unknown parameter is consistent or not. It is also applied in the context of linear regression to decide whether to choose a fixed effect model or a random effect model.
How do you choose between fixed and random effects?
The most important practical difference between the two is this: Random effects are estimated with partial pooling, while fixed effects are not. Partial pooling means that, if you have few data points in a group, the group's effect estimate will be based partially on the more abundant data from other groups.
What is fixed and random effect model?
A fixed-effect meta-analysis estimates a single effect that is assumed to be. common to every study, while a random-effects meta-analysis estimates the. mean of a distribution of effects. Study weights are more balanced under the random-effects model than under the. fixed-effect model.
What is random effects regression?
The Random Effects regression model is used to estimate the effect of individual-specific characteristics such as grit or acumen that are inherently unmeasurable. Such individual-specific effects are often encountered in panel data studies.
What is random effect model in econometrics?
In statistics, a random effects model, also called a variance components model, is a statistical model where the model parameters are random variables.
What is the meaning of endogeneity?
Endogeneity is a variable or change that arises internally from a model or system. A variable is termed endogenic when it is dependent on the other variables of the system. An exogenous variable is a variable that is not affected by other variables, but will affect other variables of the system.
What causes endogeneity?
Endogeneity may arise due to the omission of explanatory variables in the regression, which would result in the error term being correlated with the explanatory variables, thereby violating a basic assumption behind ordinary least squares (OLS) regression analysis.
How do you overcome endogeneity?
The best way to deal with endogeneity concerns is through instrumental variables (IV) techniques. The most common IV estimator is Two Stage Least Squares (TSLS). IV estimation is intuitively appealing, and relatively simple to implement on a technical level.
How do you test for endogeneity without instruments?
We cannot do endogeneity test without a valid instrument. Therefore, we have to have strong argument for a valid instrument first before we can do endogeneity test. With endogenous variables on the right-hand side of the equation, we need to use instrumental variable (IV) regression for consistent estimation.
What is the null hypothesis for a Hausman test for endogeneity?
The null hypothesis is that the preferred model is random effects; The alternate hypothesis is that the model is fixed effects. Essentially, the tests looks to see if there is a correlation between the unique errors and the regressors in the model. The null hypothesis is that there is no correlation between the two.
How do you test for endogeneity in SPSS?
1:158:02Endogeneity tests - YouTubeYouTubeStart of suggested clipEnd of suggested clipSo testing for endogeneity. Basically our boils down the testing if this correlation is nonzero ifMoreSo testing for endogeneity. Basically our boils down the testing if this correlation is nonzero if the correlation will in those two error terms is nonzero then. We have an end identity.
What is a specification test in econometrics?
In econometrics, specification tests have been constructed to verify the validity of one specification at a time. It is argued that most of these tests are not, in general, robust in the presence of other misspecifications, so their application may result in misleading conclusions.
What is Hausman test?
Hausman tests (Hausman 1978) are tests for econometric model misspecification based on a comparison of two different estimators of the model parameters. The estimators compared should have the properties that (1) under the null hypothesis of correct model specification both estimators are consistent for the “ true parameters ” of the model (those corresponding to the data generating process), whereas (2) under misspecification (the alternative hypothesis) the estimators should have differing probability limits. The former property ensures that the size of the test can be controlled asymptotically, and the latter property gives the test its power. Heuristically, the key idea is that when the model is correctly specified, the compared estimators will be close to one another, but when the model is misspecified, the compared estimators will be far apart.
How does the Hausman test work?
One performs a Hausman test by comparing the Hausman statistic to a critical value obtained from its sampling distribution, and rejecting the null hypothesis of correct specification if the Hausman statistic exceeds its critical value. The large sample distribution of the Hausman statistic is straightforward to derive; a high-level analysis appears below. This distribution simplifies usefully when one of the compared estimators is efficient under the null, as originally proposed by Jerry Hausman (1978).
What is Hausman's motivation?
Although Hausman ’ s initial motivation and examples concerned linear models and the orthogonality assumptions (e.g., independence) that are typically central to identification in econometric models, he particularly emphasizes the generality and unifying nature of the estimator comparison approach.
What is the role of S in testing partially correct specification?
In testing partially correct specification, S can also play a useful role by selecting or combining only those coefficients consistently estimated under partially correct specification.
Does the Hausman test have consistency?
An important caveat for part (ii) is the necessity of the requirement that misspecification entails . As Alberto Holly (1982) has pointed out, this can fail for particular types of misspecification in combination with particular choices of compared estimators. The Hausman statistic just given is thus not guaranteed to yield a test consistent against arbitrary model misspecification. Nevertheless, a clever modification of the Hausman statistic proposed by Herman Bierens (1988) gives a variant of the Hausman test that does have this consistency property (see also Bierens 1990).
Which test is used to test whether the IV or OLS regression technique is best?
To test whether the IV or OLS regression technique is best, one can use the Hausman endogeneity test. Let us try to estimate the following equation:
What is OLS estimation?
If you have studied basic statistics, its likely that you have come across the ordinary least squares ( OLS) estimation technique . OLS attempts to minimize the squared distance between dependent variables (‘ y ‘) and the a linear prediction of y (y_hat= xβ ). The parameter vector ‘ β_ols ‘ minimizes this distance.  The most important assumption in order for β to reflect to true parameters in the population is for the regressors to be uncorrelated with the error terms ( cov (x,e)=0 ). Sometimes this is not the case. The assumption fails if:
What happens if you reject the null hypothesis?
If one accepts the null hypothesis, then there is no endogeneity problem and OLS should be used . If one rejects the null hypothesis, then endogeneity is a problem and one should use an IV estimation strategy.
Can we estimate Î?
We can now consistently estimate δ ,α, and Ï? using OLS. Using the usual OLS t-statistic, we can test the null hypothesis that Ï?=0. If we accept the null, then there is no endogeneity problem and one should use an OLS estimation strategy. If Ï?â 0, then the instrumental variables technique is best. One can also use a heteroskedasticity-robust t-statistic for testing Ï? if one suspects heteroskedasticity.Â
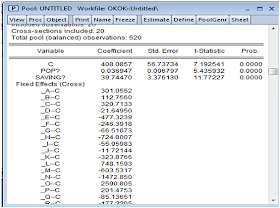