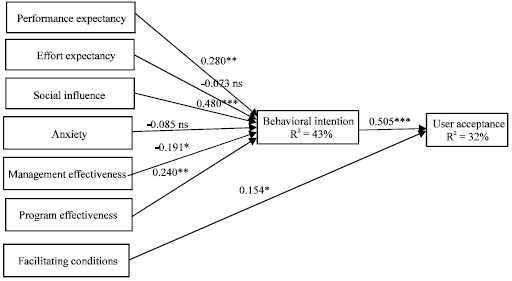
There are different probability models for continuous outcomes, and the appropriate model depends on the distribution of the outcome of interest. The normal probability model applies when the distribution of the continuous outcome conforms reasonably well to a normal or Gaussian distribution, which resembles a bell shaped curve.
How can I tell if my model has a normal distribution?
The residuals of your model (the variance not explained by your model) have to follow a normal distribution. You can check this by an histogram of the residuals or by a quantile-quantile plot. You can see on the graphs below how it should looks like when you have normality.
When do you use normal probability model?
Note normal probability model can be used even if the distribution of the continuous outcome is not perfectly symmetrical; it just has to be reasonably close to a normal or Gaussian distribution. However, other distributions do not follow the symmetrical patterns shown above.
How do you know if a model fits the data well?
If the model fit to the data were correct, the residuals would approximate the random errors that make the relationship between the explanatory variables and the response variable a statistical relationship. Therefore, if the residuals appear to behave randomly, it suggests that the model fits the data well.
How do you check normality in statistics?
You may also visually check normality by plotting a frequency distribution, also called a histogram, of the data and visually comparing it to a normal distribution (overlaid in red). In a frequency distribution, each data point is put into a discrete bin, for example (-10,-5], (-5, 0], (0, 5], etc.

How do I know if my data is normally distributed?
You may also visually check normality by plotting a frequency distribution, also called a histogram, of the data and visually comparing it to a normal distribution (overlaid in red).
What are the assumptions of the normal model?
The core element of the Assumption of Normality asserts that the distribution of sample means (across independent samples) is normal. In technical terms, the Assumption of Normality claims that the sampling distribution of the mean is normal or that the distribution of means across samples is normal.
What are the properties of a normal model?
Properties of a normal distribution The mean, mode and median are all equal. The curve is symmetric at the center (i.e. around the mean, μ). Exactly half of the values are to the left of center and exactly half the values are to the right. The total area under the curve is 1.
Why is normal distribution not a good model?
My answer: Since the standard deviation is quite large (=15.2), the normal curve will disperse wildly. Hence, it is not a good approximation.
How do you know if normality assumptions are met?
Draw a boxplot of your data. If your data comes from a normal distribution, the box will be symmetrical with the mean and median in the center. If the data meets the assumption of normality, there should also be few outliers. A normal probability plot showing data that's approximately normal.
What are the requirements for normal distribution?
Normal distributions have the following features:symmetric bell shape.mean and median are equal; both located at the center of the distribution.≈68%approximately equals, 68, percent of the data falls within 1 standard deviation of the mean.More items...
What are the 4 characteristics of a normal distribution?
Here, we see the four characteristics of a normal distribution. Normal distributions are symmetric, unimodal, and asymptotic, and the mean, median, and mode are all equal. A normal distribution is perfectly symmetrical around its center.
How do you analyze a normal distribution?
For quick and visual identification of a normal distribution, use a QQ plot if you have only one variable to look at and a Box Plot if you have many. Use a histogram if you need to present your results to a non-statistical public. As a statistical test to confirm your hypothesis, use the Shapiro Wilk test.
How do you identify the characteristics of a normal distribution?
In order to be considered a normal distribution, a data set (when graphed) must follow a bell-shaped symmetrical curve centered around the mean. It must also adhere to the empirical rule that indicates the percentage of the data set that falls within (plus or minus) 1, 2 and 3 standard deviations of the mean.
When can normal distribution not be used?
Insufficient Data can cause a normal distribution to look completely scattered. For example, classroom test results are usually normally distributed. An extreme example: if you choose three random students and plot the results on a graph, you won't get a normal distribution.
What are the limitations of normal distribution?
One of the disadvantages of using the normal distribution for reliability calculations is the fact that the normal distribution starts at negative infinity. This can result in negative values for some of the results.
Why do we want data to be normally distributed?
One reason the normal distribution is important is that many psychological and educational variables are distributed approximately normally. Measures of reading ability, introversion, job satisfaction, and memory are among the many psychological variables approximately normally distributed.
What are the assumptions of the classical normal linear regression model?
Assumptions of the Classical Linear Regression Model: The error term has a zero population mean. 3. All explanatory variables are uncorrelated with the error term 4. Observations of the error term are uncorrelated with each other (no serial correlation).
What are the assumptions of a linear model?
There are four assumptions associated with a linear regression model: Linearity: The relationship between X and the mean of Y is linear. Homoscedasticity: The variance of residual is the same for any value of X. Independence: Observations are independent of each other.
What is normality assumption in ANOVA?
So you'll often see the normality assumption for an ANOVA stated as: “The distribution of Y within each group is normally distributed.” It's the same thing as Y|X and in this context, it's the same as saying the residuals are normally distributed.
Why is normal distribution an assumption of the t tests?
The purpose of the t-test is to compare certain characteristics representing groups, and the mean values become representative when the population has a normal distribution. This is the reason why satisfaction of the normality assumption is essential in the t-test.
When do you have homogeneity?
You have homogeneity when the spread is more or less the same for all the residuals ( you do not see any particular pattern, see figure below). If your residuals show a pattern (linear or non linear) or have a cone shape (spread higher in one side of the graph and lower at the other side), this assumption is not supported ...
Can you take age as an explanatory variable?
In other words, the uncertainty on X has to be the lowest as possible. for example, you cannot take age as an explanatory variable if the lifespan is 25 years and you have an uncertainty of 3 years.
Is normality the most important assumption?
You can see on the graphs below how it should looks like when you have normality. However, normality is not the most important assumption and linear models are robust enough to a small amount of non-normality. Checking for homogeneity: This assumption is much more important.
Do you need to use colinear variables in a multivariate regression?
In the case of a multivariate linear regression, your explanatory variables have to be independent. In other words, do not use colinear variables in the same model. To check this, plot one variable against the other. If you detect a strong linear or non linear pattern, they are dependent. Once you have applied your model.
What percentage of the values fall between the mean and two standard deviations?
Approximately 95% of the values fall between the mean and two standard deviations (in either direction)
Is a bell shaped distribution symmetrical?
It is bell-shaped with a single peak in the center, and it is symmetrical. If the distribution is perfectly symmetrical with a single peak in the center, then the mean value, the mode, and the median will be all be the same. Many variables have similar characteristics, which are characteristic of so-called normal or Gaussian distributions.
Is 30 a mean or standard deviation?
Because 30 is neither the mean nor a multiple of standard deviations above or below the mean, we cannot simply use the probabilities known to be associated with 1, 2, or 3 standard deviations from the mean. In a sense, we need to know how far a given value is from the mean and the probability of having values less than this. And, of course, we would want to have a way of figuring this out not only for BMI values in a population of males with a mean of 29 and a standard deviation of 6, but for any normally distributed variable. So, what we need is a standardized way of evaluating any normally distributed data so that we can compute the probability of observing the results obtained from samples that we take. We can do all of this fairly easily by using a "standard normal distribution."
What tests can you use to check if a model is normal?
There are both visual and formal statistical tests that can help you check if your model residuals meet the assumption of normality. In Prism, most models (ANOVA, Linear Regression, etc.) include tests and plots for evaluating normality, and you can also test a column of data directly.
How to check normality of data?
You may also visually check normality by plotting a frequency distribution, also called a histogram, of the data and visually comparing it to a normal distribution (overlaid in red). In a frequency distribution, each data point is put into a discrete bin, for example (-10,-5], (-5, 0], (0, 5], etc. The plot shows the proportion of data points in each bin.
What if my residuals aren’t normally distributed?
If there is evidence your data are significantly different from the expected normal distribution, what can you do?
What is the assumption of ANOVA with fixed effects?
In two-way ANOVA with fixed effects, where there are two experimental factors such as fertilizer type and soil type, the assumption is that data within each factor combination are normally distributed. It’s easiest to test this by looking at all of the residuals at once.
What is the most common tool for assessing normality?
The most common graphical tool for assessing normality is the Q-Q plot. In these plots, the observed data is plotted against the expected quantiles of a normal distribution. It takes practice to read these plots. In theory, sampled data from a normal distribution would fall along the dotted line. In reality, even data sampled from a normal distribution, such as the example QQ plot below, can exhibit some deviation from the line.
What are the statistical tests for normality?
There are many statistical tests to evaluate normality, although we don’t recommend relying on them blindly. Prism offers four normality test options: D'Agostino-Pearson, Anderson-Darling, Shapiro-Wilk and Kolmogorov-Smirnov. Each of the tests produces a p-value that sums up the results for a researcher: 1 If the p-value is not significant, the normality test was “passed”. While it’s true we can never say for certain that the data came from a normal distribution, there is not evidence to suggest otherwise. 2 If the p-value is significant, the normality test was “failed”. There is evidence that the data may not be normally distributed after all.
Can you test if data are normal?
You can test the hypothesis that your data were sampled from a Normal (Gaussian) distribution visually (with QQ-plots and histograms) or statistically (with tests such as D'Agostino-Pearson and Kolmogorov-Smirnov). However, it’s rare to need to test if your data are normal. Most likely you’re fitting some type of statistical model to your data such as ANOVA, linear regression, and nonlinear regression. In these cases, the assumption is that the residuals, the deviations between the model predictions and the observed data, are sampled from a normally distribution. The residuals need to be approximately normally distributed to get valid statistical inference such as confidence intervals, coefficient estimates, and p values.
How to fit a normal curve to data?
Formula of the normal curve. Once you have the mean and standard deviation of a normal distribution, you can fit a normal curve to your data using a probability density function. In a probability density function, the area under the curve tells you probability.
What is the standard normal distribution?
The standard normal distribution, also called the z-distribution, is a special normal distribution where the mean is 0 and the standard deviation is 1.
What is the difference between mean and standard deviation?
The mean is the location parameter while the standard deviation is the scale parameter.
How to get a population mean?
In research, to get a good idea of a population mean, ideally you’d collect data from multiple random samples within the population. A sampling distribution of the mean is the distribution of the means of these different samples.
How many values are within 1 standard deviation from the mean?
Around 68% of values are within 1 standard deviation from the mean.
Why are normal distributions also called bell curves?
Normal distributions are also called Gaussian distributions or bell curves because of their shape.
What is empirical rule?
The empirical rule is a quick way to get an overview of your data and check for any outliers or extreme values that don’t follow this pattern.

Normal (Gaussian) Distributions
Skewed Distributions
- However, other distributions do not follow the symmetrical patterns shown above. For example, if we were to study hospital admissions and the number of days that admitted patients spend in the hospital, we would find that the distribution was not symmetrical, but skewed. Note that the distribution to the distribution below is not symmetrical, and the mean value is not the same as t…
Characteristics of Normal Distributions
- Distributions that are normal or Gaussian have the following characteristics: 1. Approximately 68% of the values fall between the mean and one standard deviation (in either direction) 2. Approximately 95% of the values fall between the mean and two standard deviations (in either direction) 3. Approximately 99.9% of the values fall between the mean ...
BMI in Males
- Consider body mass index (BMI) in a population of 60 year old males in whom BMI is normally distributed and has a mean value = 29 and a standard deviation = 6. The standard deviation gives us a measure of how spread out the observations are. The mean (μ = 29) is in the center of the distribution, and the horizontal axis is scaled in increments of the standard deviation (σ = 6) and …
Z Scores Are Standardized Scores
- We were looking at body mass index (BMI) in a population of 60 year old males in whom BMI was normally distributed and had a mean value = 29 and a standard deviation = 6. What is the probability that a randomly selected male from this population would have a BMI less than 30?" While a value of 30 doesn't fall on one of the increments of standard deviation, we can caculate …