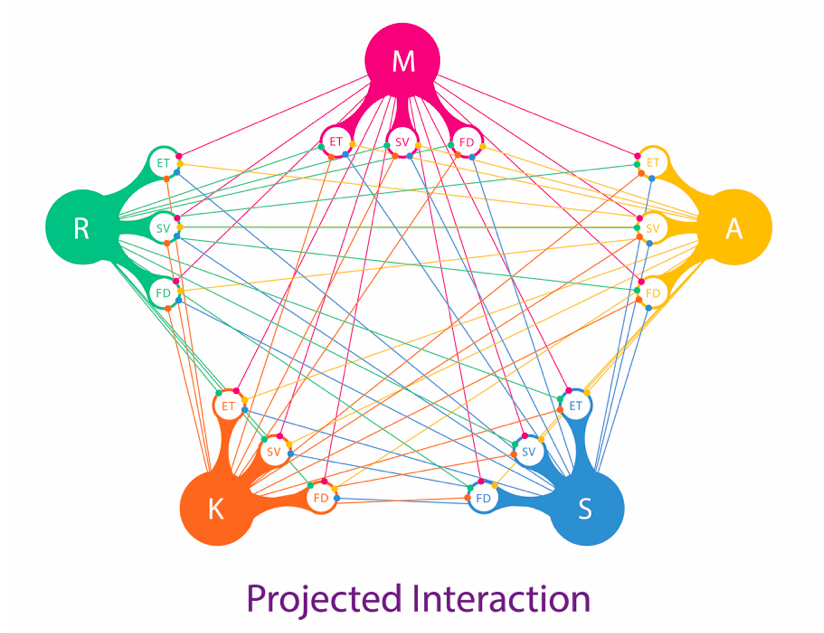
What is the difference between experience and learning in connectionism?
Learning in connectionist models is the process of connection wei ght adjustment. In contrast experience, that is, through repeated exposure to stimuli from the environment. Two broad connection weights are dependent on an error si gnal or not. In the former case, learning is network and a target response sp ecified by the environment.
What are the constraints of a connectionist model?
Connectionist models contain a number of constraints (architecture, activation dynamics, input and output representations, learning algorithm, training regime) that determine the efficiency and outcome of learning.
What is connectionism in early childhood education?
Connectionist models have simulated large varieties and amounts of developmental data while addressing important and longstanding developmental issues. Connectionist approaches provide a novel view of how knowledge is represented in children and a compelling picture of how and why developmental transitions occur.
What is connectionism in cognitive psychology?
Also known as artificial neural network (ANN) or parallel distributed processing (PDP) models, connectionism has been applied to a diverse range of cognitive abilities, including models of memory, attention, perception, action, language, concept formation, and reasoning (see, e.g., Houghton, 2005).
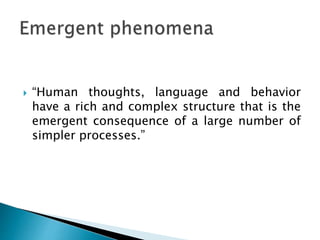
How does learning occur in connectionist models quizlet?
During learning in a connectionist network, the difference between the output signal generated by a particular stimulus and the output that actually represents that stimulus. In categorization, members of a category that a person has experienced in the past.
What are the main components of a connectionist model?
Connectionist models consist of a large number of simple processors, or units, with relatively simple input/output functions that resemble those of nerve cells. These units are connected to each other and some also to input or output structures, via a number of connections. These connections have different “weight”.
What is a connectionist approach?
Connectionist Approaches Higher-level information processing emerges from the massively-parallel interaction of these units by means of their connections, and a network may adapt its behavior by means of local changes in the strength of the connections.
What is connectionist model in cognition?
Connectionist Models in Cognitive Psychology is a state-of-the-art review of neural network modelling in core areas of cognitive psychology including: memory and learning, language (written and spoken), cognitive development, cognitive control, attention and action.
Why is connectionism important in language learning?
Because it has staked out such a wide territory, connectionism is committed to providing an account of all of the core issues in language acquisition, including grammatical development, lexical learning, phonological development, second language learning, and the processing of language by the brain.
What is the connectionist view of memory?
According to the Connectionist View, what is memory? Memories are not large knowledge structures (as in schema theories). Instead, memories are more like electrical impulses, organized only to the extent that neurons, the connections among them, and their activity are organized.
How does Hebbian learning work?
Also known as Hebb's Rule or Cell Assembly Theory, Hebbian Learning attempts to connect the psychological and neurological underpinnings of learning. The basis of the theory is when our brains learn something new, neurons are activated and connected with other neurons, forming a neural network.
What is the hidden units in connectionist model?
A hidden unit refers to the components comprising the layers of processors between input and output units in a connectionist system.
Which term is referred to as connectionist system?
1 Connectionist systems are also sometimes referred to as 'neural networks' (abbreviated to NNs) or 'artificial neural networks' (abbreviated to ANNs).
What is the unit of connectionism?
Consequently, a unit in a connectionist network is analogous to a neuron. A connection in a connectionist model is analogous to a synapse. So, just as neurons are the basic information processing structures in biological neural networks, units are the basic information processing structures in connectionist networks.
What is connectionism based on?
Connectionism was based on principles of associationism, mostly claiming that elements or ideas become associated with one another through experience and that complex ideas can be explained through a set of simple rules. But connectionism further expanded these assumptions and introduced ideas like distributed representations ...
What is the theory of connectionism?
Connectionism represents psychology's first comprehensive theory of learning 2) . It was introduced by Herbert Spencer, William James and his student Edward Thorndike in the very beginning of the 20th century although its roots date way back.
What is the practical meaning of connectivism?
Practical implications of Thorndike's ideas are suggested through his laws of learning:
What is the law of readiness?
Law of readiness. Learning is facilitated by learner's readiness (emotional and motivational) to learn. This potential to learn leads to frustration if not satisfied. This laws have set the basic principles of behaviorist stimulus-response learning, which was according to Thorndike the key form of learning.
What did Thorndike try to prove?
Thorndike tried to prove that all forms of thoughts and behaviors can be explained through S-R relations with use of repetition and reward, without need for introducing any unobservable internal states, yet this is today generally considered incorrect. This learning through response was later in 20th century replaced by learning as knowledge construction. Connectionism was in the first decades of 20th century succeeded by behaviorism, but Thorndike's experiments also inspired gestalt psychology .
What is Thorndike's book on learning mathematics 9?
In his book on learning of mathematics 9), Thorndike suggested problems children are expected to solve and learn from should be realistic. For example learning to multiply by three should be learned in context of converting feet to yards. He also emphasizes importance of repetition and insists on repetitive practice of basic arithmetic operations. Some of the principles in this book even seem inconsistent with his views on learning: here he refers to learning as meaningful and insightful. Well-learned basic skills enable learning of higher-order skills.
What are Thorndike's laws of learning?
Practical implications of Thorndike's ideas are suggested through his laws of learning: 1 rewards promote learning, but punishments do not lead to learning, 2 repetition enhances learning, and 3 potential to learn needs to be satisfied.
Why are connectionist models so popular?
Connectionist models are attractive because they appear to offer the beginnings of a computational architecture that is more neural-like. They seem to show how complex mental operations can be derived from slow, simple mechanisms, and they seem naturally to relate perception to cognition. They have had some initial successes in modeling behavior (see McClelland and Rumelhart, 1986; Rumelhart and McClelland, 1986; Smolensky, 1988 ). These issues, however, are heavily contested (see Fodor and Pylyshyn, 1988 ). Connectionist models have been most successful at pattern recognition tasks. It is not known whether they will have adequate computational power to model higher-level cognitive behavior ( Smolensky, 1988 ). At present, the state of connectionist models is changing so rapidly that detailed commentary on particular lines of research would be out of date immediately.
What is connectionist system?
In a connectionist system, different segments of a formula are represented independently within a network. Connectionism is based on neural networks that can be modeled by differential equations—they are based on finer grained microfeatures than symbols; that is, they are subsymbolic, intermediate between neural foundations and cognitive systems ( Smolensky, 1988 ). Neural networks learn from example by generalizing from input–output pairings of the training data. Knowledge is represented as numerical weights. The neural network is commonly treated as a black box in which its internal mechanism is hidden from analysis. Such neural networks do not offer transparency in that knowledge and inferencing are distributed within a network as a set of microfeatures that by themselves are not readily analyzable—it is the interrelationships between these entities that are important. Subsymbolicism implies that connectionist processing involves microfeatures that are elements distributed through the network—they are not semantically interpretable into properties of the world unless constituted with other microfeatures into symbols through patterns of activation. Higher level cognition involves symbolic processing whereby cognition emerges from lower (subsymbolic) level of neurons and neural assemblies ( Fodor and Pylyshyn, 1988 ). The neural network’s set of weights cooperate in a shared subsymbolic representation. The symbol then emerges as a collective cooperation between the distributed set of neurons. This form of representation is analogous to a hologram. Neural networks process truth values of instances to variables from which they learn a model theory ( Komendantskaya, 2009 ). They offer the ability to learn from training examples through adaptation of their connection weights. Connectionism can determine the effect of an input on the output by tracking the error terms in each step of learning. The relative contributions of each input on output errors can thus be determined over time. The chief advantage of neural networks lies in their learning ability.
What is the importance of Rumelhart and Norman's typing model?
Another important feature of Rumelhart and Norman's typing model is that it lacks a mechanism for timing, although it has a mechanism for serial ordering (inhibitory connections from “early” to “late” response nodes). At first, the absence of a timing mechanism is appealing, for generally one wants to have the most parsimonious model possible. Nevertheless, it may be that a timing mechanism is necessary. Grudin (cited in Gentner & Norman, 1984) observed that transposition errors preserve the timing of correct keystroke orders. When typists type thme instead of them, for example, the m is typed at the time that e usually occurs. A priori, one might expect e and m to be typed in the wrong order because the initiation of m happened to come a bit too early relative to the initiation of e. If this were the case, m and e would often by typed almost simultaneously, though by chance there would be a few instances in which m happened to come first. Grudin observed instead that the delays between m and e were usually as long as the delays between e and m. This surprising phenomenon suggests that timing may be independently represented in the plan for keystrokes, contrary to what Rumelhart and Norman assumed.
What are the nodes in the Rumelhart model?
In Rumelhart and Norman's model of typewriting (see Figure 8.17) distinct nodes exist for each key of the keyboard. Nodes allow for associations between fingers and keys. The node for the letter “v” associates the left index finger with the “v” key, the node for the letter “e” associates the left middle finger with the “e” key, and so on. When a decision is made to type a word such as very, the v, e, r, and y nodes are activated, along with the connections among them. The connections define the serial order of the letters. The v node inhibits the nodes for e, r, and y, the e node inhibits the nodes for r and y, and the r node inhibits the node for y. Thus this is an interelement inhibition model (see pp. 83–85 ). When a node is activated, its keystroke is initiated, but once the keystroke has been initiated, its own node is inhibited. At first, the only node that is uninhibited is v, so the v keystroke is initiated. A short time later, the v node is inhibited and so it stops inhibiting the other nodes. As a result, one node is now uninhibited— the one for e— and so the e keystroke begins. Shortly thereafter, the e node is inhibited, so it stops inhibiting the nodes for r and y. The r node is then released from inhibition, so it is initiated, and finally its self-inhibition allows the y keystroke to begin.
How does the timing relationship between keystrokes work?
Another important feature of Rumelhart and Norman’s (1982) model is that many of the timing relations between keystrokes arise entirely from the mechanical coupling of the hands and fingers ; they are not explicitly controlled by the network. If a keystroke is initiated while another keystroke is under way, its trajectory is influenced by the earlier stroke. Specifically, each keystroke proceeds more and more directly to its target the less it is mechanically coupled to the hand or finger still performing an earlier keystroke. If the keystroke being performed uses the identical finger to the keystroke before it, it undergoes a significant detour; if it uses a different finger of the same hand, it undergoes less of a detour; and if it uses the other hand, it undergoes even less of a detour. Thus, the model relies on mechanical interactions to account for the within-hand and between-hand timing effects observed in typewriting. Note that this reliance on physical properties of the system being modeled is what we have seen in other domains of human motor control, such as the analysis of walking, where important advances have been made by relying on preflexes as well as reflexes (see Chapter 5 ).
What is the timing problem with Rumelhart and Norman's model?
Another timing-related problem with Rumelhart and Norman's model is that it appears to make some wrong predictions about variations in overall typing rate. The only way to vary typing rates within Rumelhart and Norman's model is to change inhibition levels among letter nodes. When this is done, however, the simulated timing changes do not agree with those observed in real typing ( Gentner, 1987, p. 272 ). Whether this problem can be fixed without an explicit timing mechanism remains to be seen.
When are nodes self-inhibited?
In Rumelhart and Norman’s (1982) model, nodes are self-inhibited as soon as their corresponding keystrokes are initiated, but they can also be self-inhibited before their corresponding keystrokes are completed. Because the self-inhibition of a node can occur while its keystroke is still in progress, subsequent keystrokes can begin while preceding keystrokes are under way. This provides a way of explaining the simultaneous activity of hands and fingers during typewriting.
What is connectionism in psychology?
Connectionism promises to explain flexibility and insight found in human intelligence using methods that cannot be easily expressed in the form of exception free principles (Horgan & Tienson 1989, 1990), thus avoiding the brittleness that arises from standard forms of symbolic representation.
What is the purpose of connectionism?
Connectionism is a movement in cognitive science that hopes to explain intellectual abilities using artificial neural networks (also known as “neural networks” or “neural nets”). Neural networks are simplified models of the brain composed of large numbers of units (the analogs of neurons) together with weights that measure the strength ...
Why are neural networks important?
Philosophers are interested in neural networks because they may provide a new framework for understanding the nature of the mind and its relation to the brain (Rumelhart & McClelland 1986: Chapter 1). Connectionist models seem particularly well matched to what we know about neurology. The brain is indeed a neural net, formed from massively many units (neurons) and their connections (synapses). Furthermore, several properties of neural network models suggest that connectionism may offer an especially faithful picture of the nature of cognitive processing. Neural networks exhibit robust flexibility in the face of the challenges posed by the real world. Noisy input or destruction of units causes graceful degradation of function. The net’s response is still appropriate, though somewhat less accurate. In contrast, noise and loss of circuitry in classical computers typically result in catastrophic failure. Neural networks are also particularly well adapted for problems that require the resolution of many conflicting constraints in parallel. There is ample evidence from research in artificial intelligence that cognitive tasks such as object recognition, planning, and even coordinated motion present problems of this kind. Although classical systems are capable of multiple constraint satisfaction, connectionists argue that neural network models provide much more natural mechanisms for dealing with such problems.
Why do connectionists avoid recurrent connections?
Connectionists tend to avoid recurrent connections because little is understood about the general problem of training recurrent nets. However Elman (1991) and others have made some progress with simple recurrent nets, where the recurrence is tightly constrained. 2. Neural Network Learning and Backpropagation.
How is the pattern of activation set up by a net determined?
The pattern of activation set up by a net is determined by the weights, or strength of connections between the units. Weights may be either positive or negative. A negative weight represents the inhibition of the receiving unit by the activity of a sending unit. The activation value for each receiving unit is calculated according a simple activation function. Activation functions vary in detail, but they all conform to the same basic plan. The function sums together the contributions of all sending units, where the contribution of a unit is defined as the weight of the connection between the sending and receiving units times the sending unit’s activation value. This sum is usually modified further, for example, by adjusting the activation sum to a value between 0 and 1 and/or by setting the activation to zero unless a threshold level for the sum is reached. Connectionists presume that cognitive functioning can be explained by collections of units that operate in this way. Since it is assumed that all the units calculate pretty much the same simple activation function, human intellectual accomplishments must depend primarily on the settings of the weights between the units.
Why are philosophers interested in connectionism?
Philosophers have become interested in connectionism because it promises to provide an alternative to the classical theory of the mind: the widely held view that the mind is something akin to a digital computer processing a symbolic language.
What is Elman's work on nets?
Elman’s 1991 work on nets that can appreciate grammatical structure has important implications for the debate about whether neural networks can learn to master rules. Elman trained a simple recurrent network to predict the next word in a large corpus of English sentences. The sentences were formed from a simple vocabulary of 23 words using a subset of English grammar. The grammar, though simple, posed a hard test for linguistic awareness. It allowed unlimited formation of relative clauses while demanding agreement between the head noun and the verb. So for example, in the sentence
What is the connectionist model?
Connectionism is a cognitive model that grew out of a need to have a model that allowed for and built upon the interaction between the biologically coded aspects of the brain and the learned aspects that humans receive from their environment. Connectionist models focus on the concept of neural networks, where nodes or units function as neurons with many connections to other nodes. A connectionist model is based around the idea that very simple rules applied to a large set of nodes can account for rules and categories that exist in other models, such as associationist models, spreading activation models, or hierarchical models. Instead of starting out with initial categories in the brain, connectionist models allow for the emergence of categories, based on experience. The units of these models are all very simple, consisting of a weight of an activation threshold, and a weight for its interactions with other units, as well a function for determining changes of weight for correction. One of the main benefits of the connectionist model, is that it can be simulated on computers, and has been found to closely mirror effects found in humans, such as the U-shaped performance curve for learning, and similar deficiencies when nodes are removed to those found when a brain is lesioned .
Why is the connectionist model important?
An important aspect to the connectionist model is the ways that it can be used to explain the emergence of particular properties with only simple learning rules instead of requiring bioprogrammed (highly specific and genetically determined) areas of the brain such as Chomsky's Language acquisition device. There has been a push to consider the interaction between the individual and the environment as an important aspect in innateness, and that simple rules likely evolved that, because of the environment, allow very complex networks to form . Connectionist models that have been trained to learn tasks like reading have mirrored the natural progressions of individuals, and also mirror human behaviour in the ways that they process new words.. This also means that when training these networks, they have to be given similar input as what humans get if they are going to actually be used as comparison models.
What is the function of single units in connectionist models?
Karl Lashely, in an attempt to locate this by lesioning rat’s brains in the hopes of their forgetting a task they had learned, concluded that the engram did not exist, and that memories consist of neurons throughout the brain. The colloquial name "Grandmother Cell" (GMaC) is seen to be similar to this, where a particular concept is encoded, or located in a single neuron. The name comes from the idea that there could be a cell that fired at the memory of one's grandmother, and that this firing is responsible for one's remembering. The opposing view is that variations in activation over distributed neurons cause patterns, and the neurons in these patterns have particular weights associated with their firings, and this weighted pattern of activation is what would cause a memory. The localist view must be distinguished here, because it is not merely the case that if the GMaC had not fired in this pattern, then perhaps a memory of fruitcake or one's grandfather would have been remembered. Instead, it is that the GMaC is a dedicated cell for that particular idea, and will not fire as part of a pattern for another idea, or require other neurons to fire to differentiate the idea of grandmother from a different idea. This argument stems in large part from single cell recordings where particular stimuli elicited highly selective responses for particular cells. This theory is also suggested because of the possibility of the problem that is sometimes encountered in connectionist models that is called "superposition". This is when two different stimuli result in the same output pattern, although this can be achieved through different activation of preceding units.#N#A few prominent connectionists reject this notion that there are dedicated cells for particular ideas, words or concepts. The possibility that one neuron could be dedicated to an idea runs the risk of what constitutes an idea. If there were a Grandmother cell, what about a 'Grandmother's ear' cell? And if there were one, why isn't the idea of 'grandmother' made up of all these parts of her. These arguments, while certainly not definitive, show the value of distributed, weighted activation. The fact that the activation is weighted is especially important, because one heavily weighted cell could affect the whole pattern, while a highly active one could have little effect of it had no weight.
What are the arrows in the Seidenberg and McClelland model?
The arrows represent the interactions, so the hidden units activation produced an orthography and a phonology, and were re weighted based on both reproductions.
How do units interact?
Units interact along connections that have particular weights. This means that the effect of any particular unit (a) is defined by its relation with another unit (b), and cannot be reduced to anything within (a), and is not directly affected when any other connections are changed.
What are the levels of reading?
Originally, their model for reading included a number of interconnected levels: the orthographic input, the phonological output, a 'meaning' level, three hidden levels between each of these, as well as a 'content' level that was connected to the 'meaning' level. All of these levels are theoretically capable of being altered by, and altering any on that they are directly connected to. In practice, only the input and output layers, as well as one hidden layer was used. This was in large part due to the length of time that it takes to simulate parallel processes on a serial processing computer.
What is connectionist model?
Connectionist models, also known as Parallel Distributed Processing (PDP) models, are a class of computational models often used to model aspects of human perception, cognition, and behaviour, the learning processes underlying such behaviour, and the storage and retrieval of information from memory. The approach embodies a particular perspective in cognitive science, one that is based on the idea that our understanding of behaviour and of mental states should be informed and constrained by our knowledge of the neural processes that underpin cognition. While neural network modelling has a history dating back to the 1950s, it was only at the beginning of the 1980s that the approach gained widespread recognition, with the publication of two books edited by D.E. Rumelhart & J.L. McClelland (McClelland & Rumelhart, 1986; Rumelhart & McClelland, 1986), in which the basic principles of the approach were laid out, and its application to a number of psychological topics were developed. Connectionist models of cognitive processes have now been proposed in many different domains, ranging from different aspects of language processing to cognitive control, from perception to memory. Whereas the specific architecture of such models often differs substantially from one application to another, all models share a number of central assumptions that collectively characterize the “connectionist ” approach in cognitive science. One of the central features of the approach is the emphasis it has placed on mechanisms of change. In contrast to traditional computational modelling methods in cognitive science, connectionism takes it that understanding the mechanisms involved in some cognitive process should be informed by the manner in which the system changed over time as it developed and learned. Understanding such mechanisms constitutes a significant
Who assigned distributed representations in early models?
different objects. Distributed representations in early models were assigned by the modeller,
What is the central feature of cognitive science?
cognitive science. One of the central features of the approach is the emphasis it has placed on
How does computational neuroscience work?
The goal of computational cognitive neuroscience is to understand how the brain embodies the mind by using biologically based computational models comprising networks of neuronlike units. This text, based on a course taught by Randall O'Reilly and Yuko Munakata over the past several years, provides an in-depth introduction to the main ideas in the field. The neural units in the simulations use equations based directly on the ion channels that govern the behavior of real neurons, and the neural networks incorporate anatomical and physiological properties of the neocortex. Thus the text provides the student with knowledge of the basic biology of the brain as well as the computational skills needed to simulate large-scale cognitive phenomena.The text consists of two parts. The first part covers basic neural computation mechanisms: individual neurons, neural networks, and learning mechanisms. The second part covers large-scale brain area organization and cognitive phenomena: perception and attention, memory, language, and higher-level cognition. The second part is relatively self-contained and can be used separately for mechanistically oriented cognitive neuroscience courses. Integrated throughout the text are more than forty different simulation models, many of them full-scale research-grade models, with friendly interfaces and accompanying exercises. The simulation software (PDP++, available for all major platforms) and simulations can be downloaded free of charge from the Web. Exercise solutions are available, and the text includes full information on the software.
What is computational model?
class of computational models often used to model aspects of human perception, cognition, and behaviour, the learning processes underl ying such behaviour, and the storage and. retrieval of information from memory. The appr oach embodies a particular perspective in.
Is connection weight inaccessible?
mechanisms. Connection weights ar e generally assumed to be completely inaccessible to any
Is understanding mechanisms a significant feature of time?
time as it developed and learned. Understanding such mechanisms constitutes a significant
Abstract
This chapter focuses on connectionist models of reading development, and outlines several different ways in which connectionist modelling both has been, and could be, used to advance the theoretical understanding of reading development.
Keywords
These keywords were added by machine and not by the authors. This process is experimental and the keywords may be updated as the learning algorithm improves.
Suggested Further Readings
Elman, J. L., Bates, E. A., Johnson, M. H., Karmiloff-Smith, A., Parisi, D., & Plunkett, K. (1996). Rethinking innateness: A connectionist perspective on development. Cambridge, MA: MIT Press. Google Scholar
What is connectionism in cognitive theory?
Over the last twenty years, connectionist modeling has formed an influential approach to the computational study of cognition. It is distinguished by its appeal to principles of neural computation to inspire the primitives that are included in its cognitive level models. Also known as artificial neural network (ANN) or parallel distributed processing (PDP) models, connectionism has been applied to a diverse range of cognitive abilities, including models of memory, attention, perception, action, language, concept formation, and reasoning (see, e.g., Houghton, 2005). While many of these models seek to capture adult function, connectionism places an emphasis on learning internal representations. This has led to an increasing focus on developmental phenomena and the origins of knowledge. Although, at its heart, connectionism comprises a set of computational formalisms, it has spurred vigorous theoretical debate regarding the nature of cognition. Some theorists have reacted by dismissing connectionism as mere implementation of pre-existing verbal theories of cognition, while others have viewed it as a candidate to replace the Classical Computational
What is the first feature of connectionist processing?
The first feature is the set of processing units u
What is the 6th key feature of connectionist models?
8a The sixth key feature of connectionist models is the algorithm for modifying the patterns of connectivity as a function of experience. Virtually all learning rules for PDP models can be considered a variant of the Hebbian learning rule (Hebb, 1949). The essential idea is that a weight between two units should be altered in proportion to the units’ correlated activity. For example, if a unit u
What is the interactive activation model of letter perception?
The interactive activation model of letter perception illustrates two interrelated ideas. The first is that connectionist models naturally capture a graded constraint satisfaction process in which the influences of many different types of information are simultaneously integrated in determining, for example, the identity of a letter in a word. The second idea is that the computation of a perceptual representation of the current input (in this case, a word) involves the simultaneous and mutual influence of representations at multiple levels of abstraction– this is a core idea of parallel distributed processing. The interactive activation model addressed itself to a puzzle in word recognition. By the late 1970s, it had long been known that people were better at recognizing letters presented in words than letters presented in random letter sequences. Reicher (1969) demonstrated that this was not the result of tending to
What are the assumptions of the 19 process?
There were three main assumptions of the IA model: (1) perceptual processing takes place in a system in which there are several levels of processing, each of which forms a representation of the input at a different level of abstraction; (2) visual perception involves parallel processing, both of the four letters in each word and of all levels of abstraction simultaneously; (3) perception is an interactive process in which conceptually driven and data driven processing provide multiple, simultaneously acting constraints that combine to determine what is perceived. The activation states of the system were simulated by a sequence of discrete time steps. Each unit combined its activation on the previous time step, its excitatory influences, its inhibitory influences, and a decay factor to determine its activation on the next time step. Connectivity was set at unitary values and along the following principles: in each layer, mutually exclusive alternatives should inhibit each other. For each unit in a layer, it excited all units with which it was consistent and inhibited all those with which it was inconsistent in layer immediately above. Thus in Figure 2, the 1st-position W letter unit has an excitatory connection to the WEED word unit but an inhibitory connection to the SEED and FEED word units. Similarly, a unit excited all units with which it was consistent and inhibited all those with which it was inconsistent in the layer immediately below. However, in the final implementation, top-down word-to-letter inhibition and within-layer letter-to-letter inhibition were set to zero (gray arrows, Figure 2). -------------------------------- Insert Figure 2 about here --------------------------------
How are units arranged in a network?
7 the network. Typically, units are arranged into layers (e.g., input, hidden, output) and layers of units are fully connected to each other. For example, in a three-layer feedforward architecture where activation passes in a single direction from input to output, the input layer would be fully connected to the hidden layer and the hidden layer would be fully connected to the output layer. The fourth feature is a rule for propagating activation states throughout the network. This rule takes the vector a(t)of output values for the processing units sending activation and combines it with the connectivity matrix Wto produce a summed or net input into each receiving unit. The net input to a receiving unit is produced by multiplying the vector and matrix together, so that ="()=!
Is generalization contingent on similarity?
Generalization is contingent on the similarity of verbs at input. Were the verbs to be presented using an orthogonal, localist scheme (e.g., 420 units, 1 per verb), then there would be no similarity between the verbs, no blending of mappings, no generalization, and therefore no regularization of novel verbs.
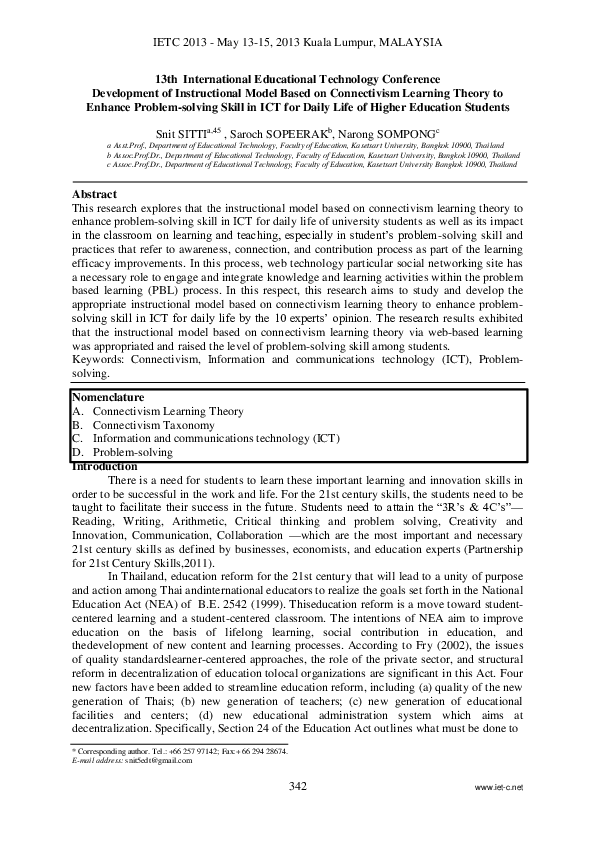
A Description of Neural Networks
Neural Network Learning and Backpropagation
- Finding the right set of weights to accomplish a given task is thecentral goal in connectionist research. Luckily, learning algorithmshave been devised that can calculate the right weights for carryingout many tasks (see Hinton 1992 for an accessible review). These fallinto two broad categories: supervised and unsupervised learning.Hebbian learning...
Samples of What Neural Networks Can Do
- Connectionists have made significant progress in demonstrating thepower of neural networks to master cognitive tasks. Here are threewell-known experiments that have encouraged connectionists to believethat neural networks are good models of human intelligence. One of themost attractive of these efforts is Sejnowski and Rosenberg’s1987 work on a net that can rea…
Strengths and Weaknesses of Neural Network Models
- Philosophers are interested in neural networks because they mayprovide a new framework for understanding the nature of the mind andits relation to the brain (Rumelhart & McClelland 1986: Chapter1). Connectionist models seem particularly well matched to what weknow about neurology. The brain is indeed a neural net, formed frommassively many units (neurons) and the…
The Shape of The Controversy Between Connectionists and Classicists
- The last forty years have been dominated by the classical view that(at least higher) human cognition is analogous to symbolic computationin digital computers. On the classical account, information isrepresented by strings of symbols, just as we represent data incomputer memory or on pieces of paper. The connectionist claims, onthe other hand, that information is stored non-s…
Connectionist Representation
- Connectionist models provide a new paradigm for understanding howinformation might be represented in the brain. A seductive but naiveidea is that single neurons (or tiny neural bundles) might be devotedto the representation of each thing the brain needs to record. Forexample, we may imagine that there is a grandmother neuron that fireswhen we think about our grandmother…
The Systematicity Debate
- The major points of controversy in the philosophical literature onconnectionism have to do with whether connectionists provide a viableand novel paradigm for understanding the mind. One complaint is thatconnectionist models are only good at processing associations. Butsuch tasks as language and reasoning cannot be accomplished byassociative methods alone and so conne…
Connectionism and Semantic Similarity
- One of the attractions of distributed representations in connectionistmodels is that they suggest a solution to the problem of providing atheory of how brain states could have meaning. The idea is that thesimilarities and differences between activation patterns alongdifferent dimensions of neural activity record semantical information.So the similarity properties of neural activations pr…
Connectionism and The Elimination of Folk Psychology
- Another important application of connectionist research tophilosophical debate about the mind concerns the status of folkpsychology. Folk psychology is the conceptual structure that wespontaneously apply to understanding and predicting human behavior.For example, knowing that John desires a beer and that he believesthat there is one in the refrigerator allows us to expl…
Predictive Coding Models of Cognition
- As connectionist research has matured from its “GoldenAge” in the 1980s, the main paradigm has radiated into a numberof distinct approaches. Two important trends worth mention arepredicative coding and deep learning (which will be covered in thefollowing section). Predictive coding is a well-establishedinformation processing tool with a wide range of applications. It isuseful, for ex…