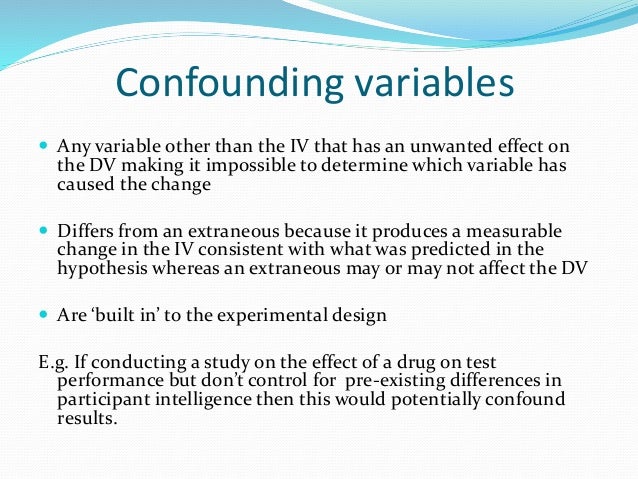
The bias resulting from the presence of a confounding variable, which obscures estimation of specific causal effects, is known as confounding bias. When confounding variables has an effect on both variables in a causal relationship of interest, the explanatory variable is associated with an outcome variable due to factors other than purely causal effects.
What is confounding bias in research paper design?
Confounding bias is bias that is the result of having confounding variables in your study design. If the observed association overestimates the effect of the independent variable on the dependent variable, this is known as positive confounding bias.
How can we predict bias when the confounding variable is omitted?
We can use correlation structures, like the one in the example, to predict the direction of bias that occurs when the model omits a confounding variable. The direction depends on both the correlation between the included and omitted independent variables and the correlation between the included independent variable and the dependent variable.
How does variable bias affect your model?
If this bias affects your model, it is a severe condition because you can’t trust your results. In this post, you’ll learn about confounding variables, omitted variable bias, how it occurs, and how to detect and correct it. What Are the Effects of Confounding Variables?
What is a confounder variable?
Confounding variables (a.k.a. confounders or confounding factors) are a type of extraneous variable that are related to a study’s independent and dependent variables. A variable must meet two conditions to be a confounder:
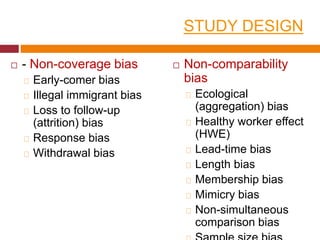
Is confounding and bias the same?
Confounding can produce either a type 1 or a type 2 error, but we usually focus on type 1 errors. Bias creates an association that is not true, but confounding describes an association that is true, but potentially misleading.
What type of bias is confounding?
Bias is an error in the estimation of an association between an exposure and an outcome due to a flaw in the design or conduct of the study. Confounding on the other hand, is a real but non-causal association between the exposure and the outcome.
What are examples of confounding variables?
Example of a confounding variable You collect data on sunburns and ice cream consumption. You find that higher ice cream consumption is associated with a higher probability of sunburn. Does that mean ice cream consumption causes sunburn?
Is experimental bias a confounding variable?
Experimenter bias is another confound that can also affect the results of an experiment. Experimenter bias happens when the experimenter's expectations influence the outcomes of the study.
What is bias in research?
In research, bias occurs when “systematic error [is] introduced into sampling or testing by selecting or encouraging one outcome or answer over others” 7. Bias can occur at any phase of research, including study design or data collection, as well as in the process of data analysis and publication (Figure 1).
How do you identify a confounding variable?
A simple, direct way to determine whether a given risk factor caused confounding is to compare the estimated measure of association before and after adjusting for confounding. In other words, compute the measure of association both before and after adjusting for a potential confounding factor.
What is a confounding variable variable?
Confounding variables are those that affect other variables in a way that produces spurious or distorted associations between two variables. They confound the "true" relationship between two variables.
What are confounders in research?
A Confounder is an extraneous variable whose presence affects the variables being studied so that the results do not reflect the actual relationship between the variables under study.
What is extraneous and confounding variable?
An extraneous variable is any variable that you're not investigating that can potentially affect the dependent variable of your research study. A confounding variable is a type of extraneous variable that not only affects the dependent variable, but is also related to the independent variable.
What is confounding bias and how can we control confounding in epidemiology?
Confounding bias: A systematic distortion in the measure of association between exposure and the health outcome caused by mixing the effect of the exposure of primary interest with extraneous risk factors.
Is gender a confounder or effect modifier?
In this case, the covariable (gender) is neither a confounder nor an effect modifier. We say that it is not a confounder because (1) the crude lies between the 2 stratum-specific estimates, but also (2) the stratum-specific estimates are not more than 10% different than the crude.
What is confounding bias?
These examples demonstrate confounding bias that occurs when an outcome is mistakenly attributed to one variable (A) when, in reality, variable (B) is responsible for the outcome. If an association between a risk factor/exposure (A) and an outcome (C) is discovered, confounding may provide an alternative explanation for the association if a third variable (B) is related to both (A) and (C). Confounding makes correlational relationships appear as causative associations. This is a major issue in observational clinical research because confounding bias can lead to spurious conclusions.
Why is confounding bias important in clinical research?
This is a major issue in observational clinical research because confounding bias can lead to spurious conclusions. A variable may be a confounding variable if it has three characteristics: The variable must be associated with the exposure/risk factor.
What is the confounding variable in the association between pretzel consumption and hepatic cirrhosis?
The confounding variable in the association between pretzel consumption and hepatic cirrhosis is beer consumption. People tend to drink more beer and eat pretzels together. When the analysis is adjusted for beer consumption , the relationship between eating pretzels and hepatic cirrhosis disappears.
When one variable creates the illusion of an association between a different variable and an outcome, this variable is considered?
When one variable creates the illusion of an association between a different variable and an outcome, this variable is considered a confounder because it distorts the true relationship between the studied variable and its outcome, leading to incorrect conclusions.
Is confounding a bad thing?
Confounding can be misleading or even dangerous. If associations are falsely attributed to one variable over another, the results may be under or overestimated. When outcomes are misrepresented, clinical decisions and recommendations will be biased and potentially inappropriate. Being mindful of possible confounders sharpens your ability to recognize potentially biased relationships and to think critically about associations that seem valid at first glance.
What is the effect of confounding variables in a statistical model?
By omitting confounding variables, the statistical procedure is forced to attribute their effects to variables in the model, which biases the estimated effects and confounds the genuine relationship. Statisticians refer to this distortion as omitted variable bias.
What Are the Effects of Confounding Variables?
Omitting confounding variables from your regression model can bias the coefficient estimates. What does that mean exactly? When you’re assessing the effects of the independent variables in the regression output, this bias can produce the following problems:
What happens if you don't take care of confounding variables?
If you aren’t careful, the hidden hazards of confounding variables and omitted variable bias can completely flip the results of your regression analysis!
What is non zero correlation?
There must be non-zero correlations (r) on all three sides of the triangle. This correlation structure causes confounding variables that are not in the model to bias the estimates that appear in your regression results. For example, removing either X variable will bias the other X variable.
What are omitted variables in regression?
Analysts often refer to omitted variables that cause bias as confounding variables, confounders, and lurking variables.
Why is my regression model biased?
This problem occurs because your linear regression model is specified incorrectly—either because the confounding variables are unknown or because the data do not exist. If this bias affects your model, it is a severe condition because you can’t trust your results.
Does omitted bias decrease correlations?
Recognize that omitted variable bias lessens as the degree of correlations decrease. It might not always be a significant problem. Understanding the relationships between the confounding variables and the variables in your model helps you make this determination.
How to reduce the impact of confounding variables
It is important to identify all possible confounding variables and consider the impact of them in your research design in order to ensure the internal validity of your results.
Frequently asked questions about confounding variables
1. What is the difference between an extraneous variable and a confounding variable?
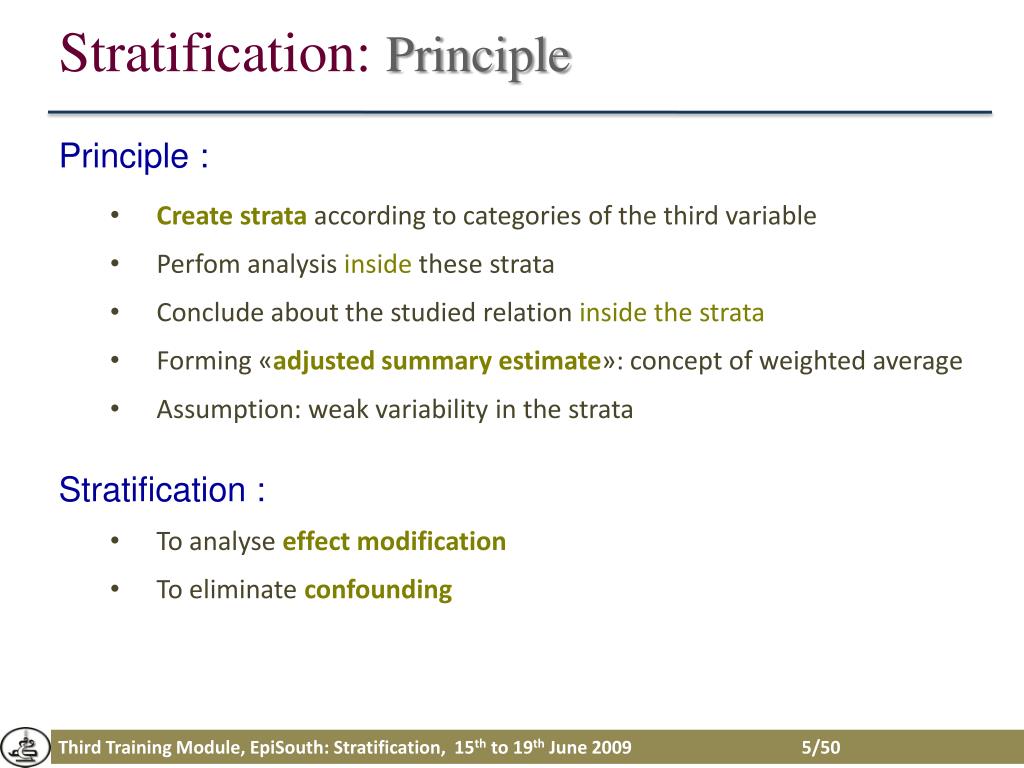