
Full Answer
What are the disadvantages of stratified random sample?
Disadvantages: Stratified Random Sampling requires more administrative works as compared with Simple Random Sampling. It is sometimes hard to classify each kind of population into clearly distinguished classes. Stratified Random Sampling can be tedious and time consuming job to those who are not keen towards handling such data.
Why and how to use stratified sampling?
- Stratification may produce a smaller error of estimation than would be produced by a simple random sample of the same size. ...
- The cost per observation in the survey may be reduced by stratification of the population elements into convenient groupings.
- Estimates of population parameters may be desired for subgroups of the population. ...
What is the difference between cluster sampling and stratified sampling?
• In cluster sampling, a cluster is selected at random, whereas in stratified sampling members are selected at random. • In stratified sampling, each group used (strata) include homogenous members while, in cluster sampling, a cluster is heterogeneous. • Stratified sampling is slower while cluster sampling is relatively faster.
What is the first step in stratified sampling?
*CHOOSING A SAMPLE*
- SIMPLE RANDOM SAMPLING – Each subject in the population has an equal chance of being selected
- STRATIFIED RANDOM SAMPLING – A representative number of subjects from various subgroups
- TWO STAGE CLUSTER RANDOM SAMPLING – Samples chosen from pre-existing groups
- SYSTEMATIC SAMPLING – Selection of every nth (i.e., 5th) subject in the population
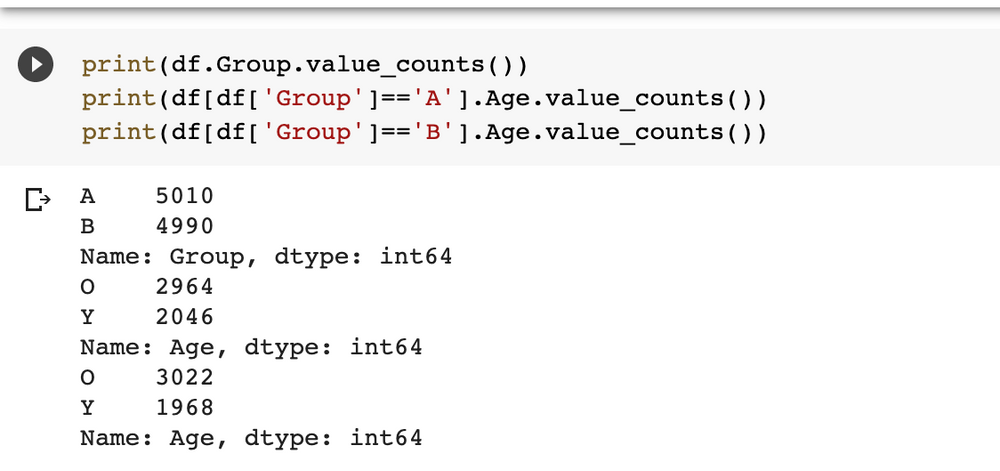
What do you mean by stratified sampling?
What is stratified sampling? In stratified sampling, researchers divide subjects into subgroups called strata based on characteristics that they share (e.g., race, gender, educational attainment). Once divided, each subgroup is randomly sampled using another probability sampling method.
What is an example of stratified sampling?
A stratified sample is one that ensures that subgroups (strata) of a given population are each adequately represented within the whole sample population of a research study. For example, one might divide a sample of adults into subgroups by age, like 18–29, 30–39, 40–49, 50–59, and 60 and above.
What does stratified mean in statistics?
Stratification consists of dividing the population into subsets (called strata) within each of which an independent sample is selected.
Why would you use stratified sampling?
Researchers use stratified sampling to ensure specific subgroups are present in their sample. It also helps them obtain precise estimates of each group's characteristics. Many surveys use this method to understand differences between subpopulations better.
When would you use a stratified sample?
Stratified random sampling is used when your population is divided into strata (characteristics like male and female or education level), and you want to include the stratum when taking your sample.
What is probability sampling?
Probability sampling means that every member of the target population has a known chance of being included in the sample. Probability sampling met...
What is stratified sampling?
In stratified sampling , researchers divide subjects into subgroups called strata based on characteristics that they share (e.g., race, gender, ed...
When should I use stratified sampling?
You should use stratified sampling when your sample can be divided into mutually exclusive and exhaustive subgroups that you believe will take on...
Can I stratify by multiple characteristics at once?
Yes, you can create a stratified sample using multiple characteristics, but you must ensure that every participant in your study belongs to one a...
What is Stratified Sampling?
Stratified sampling is a selection method where the researcher splits the population of interest into homogeneous subgroups or strata before choosing the research sample. This method often comes to play when you're dealing with a large population, and it's impossible to collect data from every member.
Why is stratified sampling important?
Stratified sampling helps you to save cost and time because you'd be working with a small and precise sample.
What is cluster sampling?
Cluster sampling involves choosing the research sample from naturally occurring groups known as clusters. In stratified sampling, the researcher selects the sample population from non-overlapping, homogeneous strata.
What is the difference between stratified and cluster sampling?
The major difference between stratified sampling and cluster sampling is how subsets are drawn from the research population. In cluster sampling, the researcher depends on naturally-occurring divisors like geographical location, school districts, and the like.
What is a disproportionate stratified sampling?
Disproportionate stratified sampling is a stratified sampling method where the sample population is not proportional to the distribution within the population of interest. The implication is that the members of different subgroups do not have an equal opportunity to be a part of the research sample.
What is the purpose of sampling fraction?
Typically, the researcher derives a sampling fraction and uses this fraction to determine how the variables are selected for the sample. This sampling fraction is always the same across all strata, regardless of their sizes. With disproportionate stratified sampling, every unit in a stratum stands the same chance of getting selected for the systematic investigation.
What are the advantages of disproportionate sampling?
A key advantage of disproportionate sampling is it allows you to collect responses from minority subsets whose sample size would otherwise be too low to allow you to draw any statistical conclusions.
When to use stratified sampling?
Description: Stratified sampling is a common sampling technique used by researchers when trying to draw conclusions from different sub-groups or strata. The strata or sub-groups should be different and the data should not overlap. While using stratified sampling, the researcher should use simple probability sampling. The population is divided into various subgroups such as age, gender, nationality, job profile, educational level etc. Stratified sampling is used when the researcher wants to understand the existing relationship between two groups.
What are some examples of SBU?
The best example of SBU are companies like Proctor and Gamble, LG etc. These companies have different product categories under one roof. For example, LG as a company makes consumer durables. It makes refrigerators, washing machines, air-conditioners as well as televisions.
Can a researcher use fractions for different subgroups?
The researcher could use different fractions for various subgroups depending on the type of research or conclusion he wants to derive from the population . The only disadvantage to that is the fact that if the researcher lays too much emphasis on one subgroup, the result could be skewed. PREV DEFINITION.
Why use stratified sampling?
The reasons to use stratified sampling rather than simple random sampling include. If measurements within strata have lower standard deviation (as compared to the overall standard deviation in the population), stratification gives smaller error in estimation.
Why is stratified sampling not useful?
Stratified sampling is not useful when the population cannot be exhaustively partitioned into disjoint subgroups. It would be a misapplication of the technique to make subgroups' sample sizes proportional to the amount of data available from the subgroups, rather than scaling sample sizes to subgroup sizes (or to their variances, if known to vary significantly—e.g. by means of an F Test ). Data representing each subgroup are taken to be of equal importance if suspected variation among them warrants stratified sampling. If subgroup variances differ significantly and the data needs to be stratified by variance, it is not possible to simultaneously make each subgroup sample size proportional to subgroup size within the total population. For an efficient way to partition sampling resources among groups that vary in their means, variance and costs, see "optimum allocation" . The problem of stratified sampling in the case of unknown class priors (ratio of subpopulations in the entire population) can have deleterious effect on the performance of any analysis on the dataset, e.g. classification. In that regard, minimax sampling ratio can be used to make the dataset robust with respect to uncertainty in the underlying data generating process.
Why is stratification important in statistics?
The strata should define a partition of the population. That is, it should be collectively exhaustive and mutually exclusive: every element in the population must be assigned to one and only one stratum. Then simple random sampling is applied within each stratum. The objective is to improve the precision of the sample by reducing sampling error. It can produce a weighted mean that has less variability than the arithmetic mean of a simple random sample of the population.
When it is desirable to have estimates of population parameters for groups within the population - stratified sampling?
When it is desirable to have estimates of population parameters for groups within the population - stratified sampling verifies we have enough samples from the strata of interest.
Why combine substrata?
Combining sub-strata to ensure adequate numbers can lead to Simpson's paradox, where trends that actually exist in different groups of data disappear or even reverse when the groups are combined.
How does stratified sampling work?
The stratified sampling process starts with researchers dividing a diverse population into relatively homogeneous groups called strata, the plural of stratum. Then, they draw a random sample from each group (stratum) and combine them to form their complete sample.
Why do we use stratified sampling?
Researchers use stratified sampling to ensure specific subgroups are present in their sample. It also helps them obtain precise estimates of each group’s characteristics. Many surveys use this method to understand differences between subpopulations better. Stratified sampling is also known as stratified random sampling.
Why do we need a random sample?
While we want a random sample for unbiased estimates overall, we also want to obtain precise estimates for each income level in our population. Using simple random sampling, income levels with a small number of students and random chance could conspire to provide small sample sizes for some income levels. These smaller sample sizes produce relatively imprecise estimates for them.
What is proportionate stratified sampling?
In proportionate stratified sampling, the sample size of each stratum is proportional to its share in the population. For example, if the rural subgroup comprises 40 percent of the population you’re studying, your sampling process will ensure it makes up 40% of the sample.
How to stratify a population?
Stratified sampling involves multiple steps. First, break down the population into strata. From each stratum, use simple random sampling to draw a sample. This process ensures that you obtain observations for all strata.
What is strata in statistics?
Strata are subpopulations whose members are relatively similar to each other compared to the broader population. Researchers can create strata based on income, gender, and race, among many other possibilities. For example, if your research question requires you to compare outcomes between income levels, you might base the strata on income. All members of the population should be in only one stratum.
How many students are selected for stratified sampling?
To avoid this problem, we’ll use stratified sampling. Our sampling plan might dictate that we select 100 students from each income level using simple random sampling. Of course, this plan presupposes that we know the household income level for each student, which might be problematic.
Which is more robust, simple random sampling or stratified random sampling?
Overall, simple random sampling is more robust than stratified random sampling, especially when a population has too many differences to be categorized.
What is the best way to select a small sample?
One of the ways researchers use to select a small sample is called stratified random sampling. Estimates generated within strata are more precise than those from random sampling because dividing the population into homogenous groups often reduces sampling error and increases precision. When seeking a potential stratum, ...
Why is sampling preferred in heterogeneous populations?
The sampling technique is preferred in heterogeneous populations because it minimizes selection bias and ensures that the entire population group is represented. It is not suitable for population groups with few characteristics that can be used to divide ...
What is sample selection bias?
Sample Selection Bias Sample selection bias is the bias that results from the failure to ensure the proper randomization of a population sample. The flaws of the sample selection
What is a simple random sample?
Simple Random Sample A simple random sample is selecting a subgroup of a population where the prospect of getting selected is equal for all the members of the population.
What is statistical significance?
Statistical Significance Statistical significance is the claim that the results or observations from an experiment are due to an underlying cause, rather than chance.
Why is a population being studied in a survey organized into groups with the same features?
A population being studied in a survey may be too large to be analyzed individually; hence, it is organized into groups with the same features to save costs and time. The technique offers wide usage, such as estimating the income for varying populations, polling of elections, and life expectancy.
What is stratified random sampling?
Stratified random sampling is a method of sampling, which is when a researcher selects a small group as a sample size for study. ...
Why is stratified random sampling more advantageous?
As a result, stratified random sampling is more advantageous when the population varies widely since it helps to better organize the samples for study.
Why is a simple random sample better than a subgroup?
However, a simple random sample is more advantageous when the population can't be organized into subgroups because there are too many differences within the population. Also, simple random samples are best when there's little-to-no information about the population, which prevents the population from being broken into subsets based on characteristics or traits.
What is a simple random sample?
A simple random sample is a sample of individuals that exist in a population whereby the individuals are randomly selected from the population and placed into the sample. This method of randomly selecting individuals seeks to select a sample size that is an unbiased representation of the population. However, a simple random sample is not advantageous when the samples of the population vary widely.
What is stratified sample?
A stratified sample can guard against an "unrepresentative" sample – for instance ending up with an all-Caucasian sample from a multi-racial population.
What is stratified random sampling?
Stratified random sampling is a form of probability sampling that provides a methodology for dividing a population into smaller subgroups as a means of ensuring greater accuracy of your high-level survey results.
What is proportionate sampling?
In proportionate sampling, the sample size of each stratum is equal to the subgroup’s proportion in the population as a whole.
What is the difference between a simple random sample and a stratified random sample?
Both are statistical measurement tools, but a simple random sample is used to represent the entire data population compared to stratified random sample, which divides the population into smaller groups based on shared characteristics.
Why is stratified sampling important?
Additionally, it makes sense to turn to stratified sampling as an efficient means to gain unique insight regarding a specific group or groups within a larger population. This will provide greater understanding of a group’s preferences and behaviors as well potentially laying the foundation for communicating with them more effectively.
What could analysis of the resulting data from respondents sorted by subgroups reveal?
Analysis of the resulting data from respondents sorted by subgroups could reveal relevant eating habits and food preferences from each identified age group. That, in turn, could help inform future menu additions aimed at getting a younger crowd through your doors or drive-thru.
What happens if you have overlap in your sample?
The result could be a misrepresentation or inaccurate reflection of the population, which is precisely what you set out to avoid in the first place.
What is stratified sampling?
In stratified sampling, the population is partitioned into non-overlapping groups, called strata and a sample is selected by some design within each stratum. For example, geographical regions can be stratified into similar regions by means of some known variables such as habitat type, elevation or soil type.
Why do we use stratified random sampling?
The principal reasons for using stratified random sampling rather than simple random sampling include: Stratification may produce a smaller error of estimation than would be produced by a simple random sample of the same size. This result is particularly true if measurements within strata are very homogeneous.
What is the objective of stratification?
If your only objective of stratification is to produce estimators with small variances, then we want to stratify such that within each stratum, the units are as similar as possible. In a survey of human population, stratification may be based on socioeconomic factors or geographic regions.
Can you stratify a key variable?
Sometimes, we would like to stratify on a key variable but cannot place the units into their correct strata until the units are sampled. For instance, in a telephone interview the respondents can not be placed into a male or female stratum until after the respondent is contacted.
When is poststratification appropriate?
Poststratification (stratification after the sample has been selected by simple random sampling) is often appropriate when a simple random sample is not properly balanced by the representation.
Does stratified random sampling always produce an estimator with a smaller variance than simple random sampling?
It is not true that stratified random sampling always produces an estimator with a smaller variance than that from simple random sampling.
Why is stratified random sampling used?
Stratified random sampling is one common method that is used by researchers because it enables them to obtain a sample population that best represents the entire population being studied, making sure that each subgroup of interest is represented. All the same, this method of research is not without its disadvantages.
How is stratified random sampling different from simple random sampling?
Stratified random sampling is different from simple random sampling, which involves the random selection of data from the entire population so that each possible sample is equally likely to occur. In contrast, stratified random sampling divides the population into smaller groups, or strata, based on shared characteristics. A random sample is taken from each stratum in direct proportion to the size of the stratum compared to the population.
Can statistical sampling be used in every study?
This method of statistical sampling, however, cannot be used in every study design or with every data set.
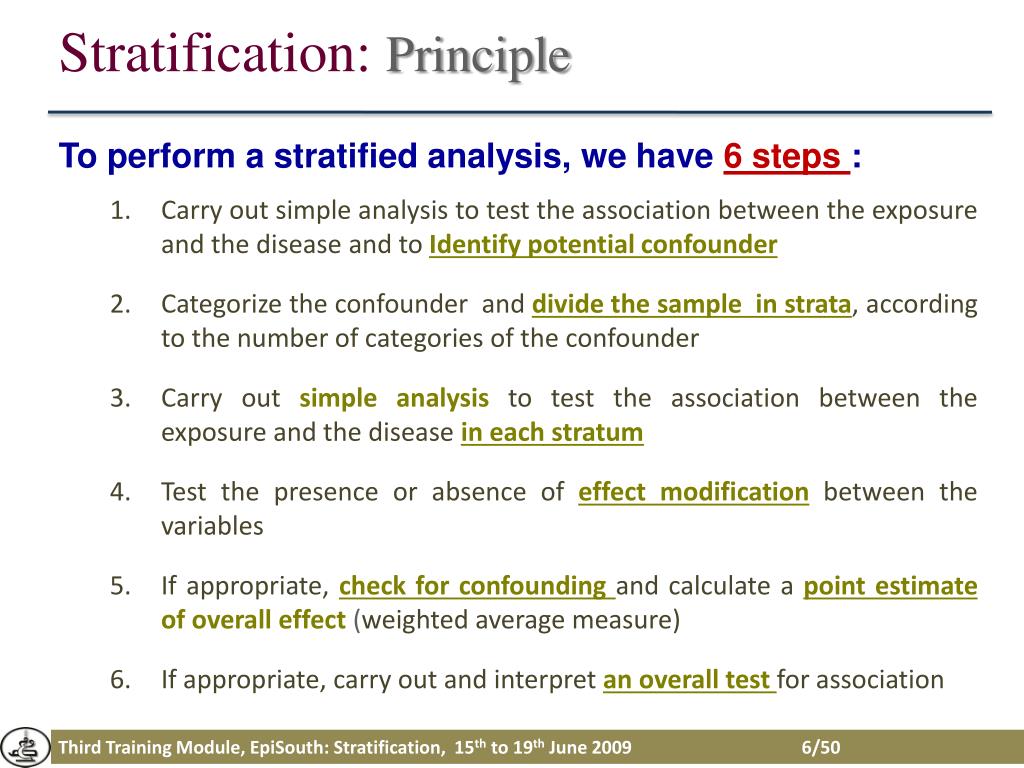
Overview
Sample size allocation
For proportional allocation strategy, the size of the sample in each stratum is taken in proportion to the size of the stratum. Suppose that in a company there are the following staff:
• male, full-time: 90
• male, part-time: 18
• female, full-time: 9
Example
Assume that we need to estimate the average number of votes for each candidate in an election. Assume that a country has 3 towns: Town A has 1 million factory workers, Town B has 2 million office workers and Town C has 3 million retirees. We can choose to get a random sample of size 60 over the entire population but there is some chance that the resulting random sample is poorly balanced across these towns and hence is biased, causing a significant error in estimation (whe…
Stratified sampling strategies
1. Proportionate allocation uses a sampling fraction in each of the strata that is proportional to that of the total population. For instance, if the population consists of n total individuals, m of which are male and f female (and where m + f = n), then the relative size of the two samples (x1 = m/n males, x2 = f/n females) should reflect this proportion.
2. Optimum allocation (or disproportionate allocation) - The sampling fraction of each stratum is proportionate to both the proportion (as a…
Advantages
The reasons to use stratified sampling rather than simple random sampling include
1. If measurements within strata have lower standard deviation (as compared to the overall standard deviation in the population), stratification gives smaller error in estimation.
2. For many applications, measurements become more manageable and/or cheaper when the population is grouped into strata.
Disadvantages
Stratified sampling is not useful when the population cannot be exhaustively partitioned into disjoint subgroups. It would be a misapplication of the technique to make subgroups' sample sizes proportional to the amount of data available from the subgroups, rather than scaling sample sizes to subgroup sizes (or to their variances, if known to vary significantly—e.g. by means of an F Test). Data representing each subgroup are taken to be of equal importance if suspected variati…
See also
• Opinion poll
• Multistage sampling
• Statistical benchmarking
• Stratified sample size
• Stratification (clinical trials)
Further reading
• Särndal, Carl-Erik; et al. (2003). "Stratified Sampling". Model Assisted Survey Sampling. New York: Springer. pp. 100–109. ISBN 0-387-40620-4.