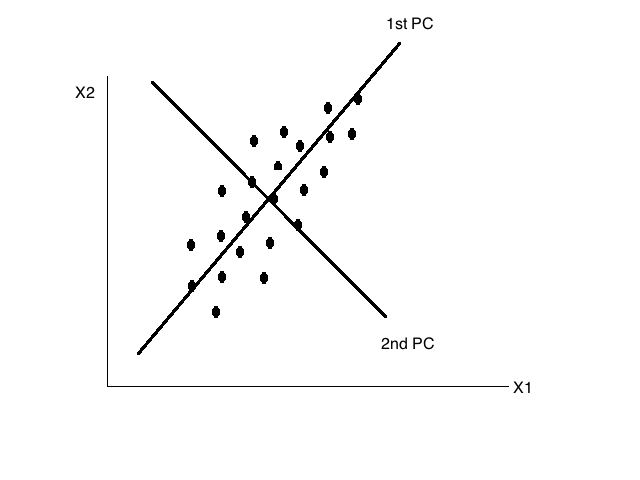
How much do all eigenvalues get shifted up?
So all the eigenvalues get shifted up by exactly 3.
What are the centers of the circles containing the eigenvalues?
There the centers of the circles containing the eigenvalues are the diagonal elements. You can always add "enough" to the diagonal element to make all the circles in the positive real half-plane. That result is more general and not needed for this. Share. Improve this answer.
Why does the least squares solution in LaSSO not touch the axis?
It is said that because the shape of the constraint in LASSO is a diamond, the least squares solution obtained might touch the corner of the diamond such that it leads to a shrinkage of some variable. However, in ridge regression, because it is a circle, it will often not touch the axis. I could not understand why it cannot touch ...
Is mathbf a positive definite matrix?
Note that the matrix $mathbf{X}^Tmathbf{X}$ is a positive definite symmetric matrix. Note that all symmetric matrices with real values have real eigenvalues. Also since it is positive definite, the eigenvalues are all greater than zero.
Is ridge regression harder than lasso?
I took ridge regression as an example, because that is much easier to treat. The lasso is much harder and there is still active ongoing researchon that topic.
Why is ridge regression hard to interpret?
Since some predictors will get shrunken very close to zero, this can make it hard to interpret the results of the model. In practice, ridge regression has the potential to produce a model that can make better predictions compared to a least squares model but it is often harder to interpret the results of the model.
What is the benefit of ridge regression?
The biggest benefit of ridge regression is its ability to produce a lower test mean squared error (MSE) compared to least squares regression when multicollinearity is present .
Why is multicollinearity a problem?
However, when the predictor variables are highly correlated then multicollinearity can become a problem. This can cause the coefficient estimates of the model to be unreliable and have high variance.
What variables are used in a linear regression model?
In ordinary multiple linear regression, we use a set of p predictor variables and a response variable to fit a model of the form:
What should be the standard deviation of a ridge regression?
Before performing ridge regression, we should scale the data such that each predictor variable has a mean of 0 and a standard deviation of 1. This ensures that no single predictor variable is overly influential when performing ridge regression.
What language is used to perform ridge regression?
The following tutorials explain how to perform ridge regression in R and Python, the two most common languages used for fitting ridge regression models:
When does shrinkage penalty become more influential?
When λ = 0, this penalty term has no effect and ridge regression produces the same coefficient estimates as least squares. However, as λ approaches infinity, the shrinkage penalty becomes more influential and the ridge regression coefficient estimates approach zero.
What is Ridge Regression?
Ridge regression shrinks all regression coefficients towards zero; the lasso tends to give a set of zero regression coefficients and leads to a sparse solution.
What is the constraint of ridge regression?
For p = 2, the constraint in ridge regression corresponds to a circle, ∑ j = 1 p β j 2 < c.
What does a colored line mean in a regression graph?
The colored lines are the paths of regression coefficients shrinking towards zero. If we draw a vertical line in the figure, it will give a set of regression coefficients corresponding to a fixed λ. (The x-axis actually shows the proportion of shrinkage instead of λ ).
What does it mean when a factor is greater than one?
If this factor is more than one, this means that ridge regression gives, on average, more squared loss as compared to linear regression. In other words, if this factor is greater than one then ridge regression is not doing a good job . This factor depends on a lot of things.
Why is a ridge solution hard to interpret?
A ridge solution can be hard to interpret because it is not sparse (no β 's are set exactly to 0). What if we constrain the L 1 norm instead of the Euclidean ( L 2 norm?
When to treat a set of regressors as a group?
In some contexts, we may wish to treat a set of regressors as a group, for example, when we have a categorical covariate with more than two levels. The grouped lasso Yuan and Lin (2007) addresses this problem by considering the simultaneous shrinkage of (pre-defined) groups of coefficients.
When fitting linear shrinkage/regularization models (ridge and lasso), should the predictors,?
When fitting linear shrinkage/regularization models (ridge and lasso), the predictors, X, should be standardized (for each predictor subtract the mean and then divide by the standard deviation). For a brand-new X, the prediction model is
What is Ridge Regression?
Ridge regression shrinks all regression coefficients towards zero; the lasso tends to give a set of zero regression coefficients and leads to a sparse solution.
Why is a ridge solution hard to interpret?
A ridge solution can be hard to interpret because it is not sparse (no β 's are set exactly to 0). What if we constrain the L 1 norm instead of the Euclidean ( L 2 norm?
What does a colored line mean in a regression graph?
The colored lines are the paths of regression coefficients shrinking towards zero. If we draw a vertical line in the figure, it will give a set of regression coefficients corresponding to a fixed λ. (The x-axis actually shows the proportion of shrinkage instead of λ ).
What is the function of lasso shrinkage?
The lasso performs L 1 shrinkage so that there are "corners'' in the constraint, which in two dimensions corresponds to a diamond. If the sum of squares "hits'' one of these corners, then the coefficient corresponding to the axis is shrunk to zero.
What is the average shrinkage of the least squares coefficients?
If, for example, c = c 0 / 2 the average shrinkage of the least squares coefficients is 50%. If λ is sufficiently large, some of the coefficients are driven to zero, leading to a sparse model.
When to treat a set of regressors as a group?
In some contexts, we may wish to treat a set of regressors as a group, for example, when we have a categorical covariate with more than two levels. The grouped lasso Yuan and Lin (2007) addresses this problem by considering the simultaneous shrinkage of (pre-defined) groups of coefficients.
Does a lasso shrink?
Hence, the lasso performs shrinkage and (effectively) subset selection.
