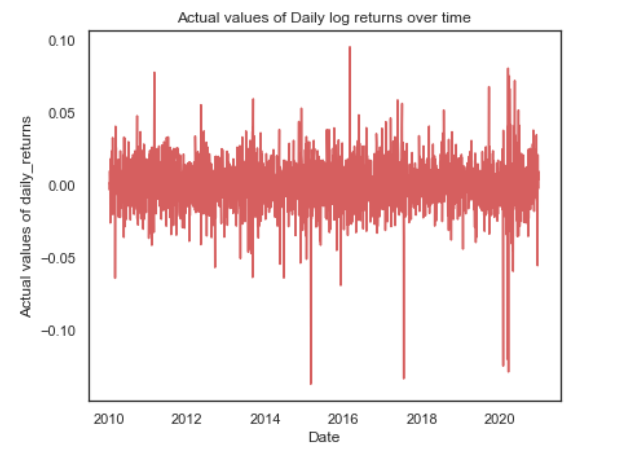
- The first conclusion of the Central Limit Theorem is the fact that the distribution of sample means is normal. This is...
- The second conclusion of the Central Limit Theorem states that the mean of the sample means is the same as the mean of...
- The third conclusion of the Central Limit Theorem states that the standard deviation of the distribution of sample means...
How do you calculate the central limit theorem?
· What are the conclusions of the Central Limit Theorem? The first conclusion the Central Limit Theorem provides is that the sampling distribution of a statistic is guaranteed to be approximately a normal distribution (provided our sample size is sufficiently large). Click to see full answer. Keeping this in view, what is the central limit theorem and why is it important in …
What is so important about the central limit theorem?
· The central limit theorem states that the sampling distribution of a sample mean is approximately normal if the sample size is large enough, even if the population distribution …
How to understand the central limit theorem?
· The central limit theorem concerns the sampling distribution of the sample means. We may ask about the overall shape of the sampling distribution. The central limit …
What does the central limit theorem enable researchers to do?
· Understanding the Central Limit Theorem Building an intuition for the most important statistical result for data science. Underlying every poll you see on the news or every …
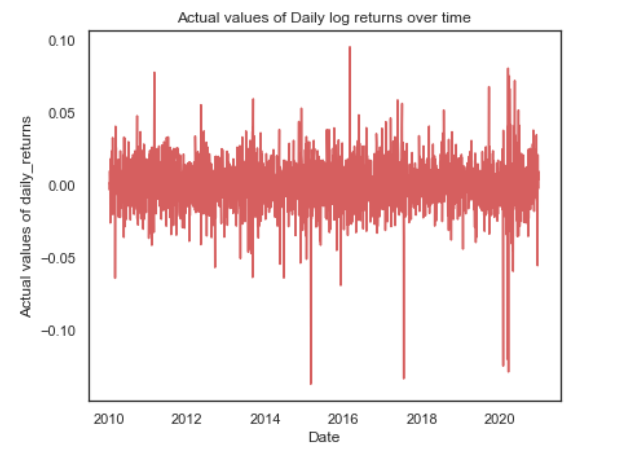
What does the central limit theorem prove?
The central limit theorem states that whenever a random sample of size n is taken from any distribution with mean and variance, then the sample mean will be approximately normally distributed with mean and variance. The larger the value of the sample size, the better the approximation to the normal.
What are the three parts of the central limit theorem?
To wrap up, there are three different components of the central limit theorem:Successive sampling from a population.Increasing sample size.Population distribution.
What is the main result from the central limit theorem?
The Central Limit Theorem states that the sampling distribution of the sample means approaches a normal distribution as the sample size gets larger — no matter what the shape of the population distribution. This fact holds especially true for sample sizes over 30.
What is not a conclusion of the central limit theorem?
The distribution of the sample data will approach a normal distribution as the sample size increases is not a conclusion of central limit theorem.
What is the central limit theorem and why is it important?
The Central Limit Theorem tells us that as sample sizes get larger, the sampling distribution of the mean will become normally distributed, even if the data within each sample are not normally distributed. We can see this in real data.
What is the central limit theorem quizlet?
The central limit theorem states that the sampling distribution of any statistic will be normal or nearly normal, if the sample size is large enough.
Why is the central limit theorem important in statistics quizlet?
The central limit theorem is important in Statistics because it: enables reasonably accurate probabilities to be determined for events involving the sample average when the sample size is large regardless of the distribution of the variable.
Why is the central limit theorem important for hypothesis testing?
Why is central limit theorem important? The central limit theorem tells us that no matter what the distribution of the population is, the shape of the sampling distribution will approach normality as the sample size (N) increases.
Why is the central limit theorem so important to the study of sampling distributions?
The central limit theorem is known to be important to the study of sampling distributions because it enables us to disregard the shape of the population when the value of n is relatively large.
Is it correct to conclude that the sample means Cannot be treated as being from a normal distribution because the sample size is too small?
Is it correct to conclude that the sample means cannot be treated as being from a normal distribution because the sample size is too small? Explain. No; the original population is normally distributed, so the sample means will be normally distributed for any sample size.
Which of the following are properties of the Student's t distribution?
Properties : The Student t distribution is different for different sample sizes. The Student t distribution is generally bell-shaped, but with smaller sample sizes shows increased variability (flatter). In other words, the distribution is less peaked than a normal distribution and with thicker tails.
What would produce a negative z score?
A negative z-score reveals the raw score is below the mean average. For example, if a z-score is equal to -2, it is 2 standard deviations below the mean. Another way to interpret z-scores is by creating a standard normal distribution (also known as the z-score distribution or probability distribution).
What is the central limit theorem?
In probability theory, the central limit theorem (CLT) states that the distribution of a sample variable approximates a normal distribution (i.e., a “bell curve”) as the sample size becomes larger, assuming that all samples are identical in size, and regardless of the population's actual distribution shape.
Who developed the Central Limit Theorem?
A sufficiently large sample size can predict the characteristics of a population accurately. Although this concept was first developed by Abraham de Moivre in 1733, it wasn’t formally named until 1930, when noted Hungarian mathematician George Polya officially dubbed it the Central Limit Theorem. 1 2 . 1:22.
Why is CLT important?
The CLT is useful when examining the returns of an individual stock or broader indices, because the analysis is simple, due to the relative ease of generating the necessary financial data. Consequently, investors of all types rely on the CLT to analyze stock returns, construct portfolios, and manage risk.
How many randomly selected stocks should be sampled for central limit theorem?
In this scenario, that investor may simply study a random sample of stocks to cultivate estimated returns of the total index. To be safe, use at least 30-50 randomly selected stocks across various sectors, should be sampled for the central limit theorem to hold. Furthermore, previously selected stocks must be swapped out with different names to help eliminate bias.
What does CLT mean?
The central limit theorem (CLT) states that the distribution of sample means approximates a normal distribution as the sample size gets larger. Sample sizes equal to or greater than 30 are considered sufficient for the CLT to hold.
Is the mean of a sample of data closer to the mean of the overall population?
According to the central limit theorem, the mean of a sample of data will be closer to the mean of the overall population in question, as the sample size increases, notwithstanding the actual distribution of the data. In other words, the data is accurate whether the distribution is normal or aberrant. As a general rule, sample sizes equal to ...
What is the central limit theorem?
The central limit theorem states that the sampling distribution of a sample mean is approximately normal if the sample size is large enough, even if the population distribution is not normal.
What is the sample mean of the central limit theorem?
Recall that the central limit theorem states that the sampling distribution of a sample mean is approximately normal if the sample size is “large enough”, even if the population distribution is not normal. If the population distribution is symmetric, sometimes a sample size as small as 15 is sufficient.
What is the sample size of a symmetric population?
If the population distribution is symmetric, sometimes a sample size as small as 15 is sufficient.
How large of a sample is sufficient for the central limit theorem?
There is no exact definition for how large a sample size needs to be in order for the central limit theorem to apply, but in general it depends on the skewness of the population distribution that the sample comes from: If the population distribution is symmetric, sometimes a sample size as small as 15 is sufficient.
What is the variance of a chi square distribution?
The variance of a chi-square distribution is 2 * df. In this case, σ2 = 2 * 3 = 6.
What does the Central Limit Theorem say about the distribution of data?
Of course, it’s important to remember that the Central Limit Theorem only says that the sample means of the data will be normally distributed. It doesn’t make any similar assumptions about the distribution of the underlying data. In other words, it doesn’t claim that the age of all the students will be normally distributed as well.
When was the Central Limit Theorem discovered?
Early version of the Central Limit Theorem was discovered as far back as 1733 by Abraham de Moivre. Today, it continues to be applied to a great extent, especially in data science and machine learning algorithms.
What is the area of the distribution on the right of the blue line?
The area of the distribution on the right of the blue line refers to the probability of observing a data point of 8.2 minutes when the true average is 7 minutes. Since this probability is sufficiently small, it is safe to say that it is extremely likely that the overall time spent on the homepage is indeed more than 7 minutes.
What is the null distribution?
According to the Central Limit Theorem, the null distribution will be a normal distribution. The theorem also states that you can use values from the sample to approximate the values needed to create the null distribution. The middle of the null distribution is the mean of the null hypothesis.
What is the standard deviation of a null distribution?
Similarly, the standard deviation of the null distribution is the standard deviation of the sample divided by the square root of the sample size.
What happens to the value of n as the sample size goes up?
As the value of n, which is the sample size, goes up. As n increases, the normal distribution is reached very quickly. Many people tend to confuse n as the number of samples. It needs to be remembered that it is, in fact, the number of data points in each sample.
What would happen if you had a number of large samples and plotted their mean?
Therefore, if you had a number of large samples and plotted their means, it would be a bell-shaped curve as seen in the graph above. In other words, most of the sample means would be closer to the center with a few averages lying on either extreme.
What is the central limit theorem?
The central limit theorem is a result from probability theory. This theorem shows up in a number of places in the field of statistics. Although the central limit theorem can seem abstract and devoid of any application, this theorem is actually quite important to the practice of statistics. So what exactly is the importance ...
Is the assumption that data is from a normal distribution unrealistic?
The assumption that data is from a normal distribution simplifies matters but seems a little unrealistic. Just a little work with some real-world data shows that outliers, skewness, multiple peaks and asymmetry show up quite routinely. We can get around the problem of data from a population that is not normal. The use of an appropriate sample size and the central limit theorem help us to get around the problem of data from populations that are not normal.
What are the two statistical conclusions?
Underlying every poll you see on the news or every estimate of an effect size in a scientific study a re two statistical conclusions: the Law of Large Numbers and the Central Limit Theorem. One of these, the Law of Large Numbers, usually strikes people as being fairly intuitive, but the other is a little harder to wrap your head around. It’s a subtle conclusion and its practical uses are non-obvious, yet it might be the single most important piece of statistics for applied data science. It underpins how statisticians and data scientists quantify margins of error, how they test to see if an effect is significant and why normal distributions (those classic, bell curve like distributions) are so common in real world variables. If you work with applied statistics in basically any capacity and want to understand why your tools work the way they do, you first need to understand the Central Limit Theorem.
What does the CLM tell us about the distribution of the sample average?
Instead, the CLM tells us something interesting about the distribution of the sample average. Remember that a single measurement in our survey was a random variable. The sample average is also a random variable, since it is entirely derived from the measurements, which were themselves random variables. Because of the CLM, we know something important about the shape of the distribution of the sample average — namely, that as the sample size grows, it becomes normally distributed.
What is normal distribution?
Normal distribution: the normal distribution is a class of distributions that people tend to be familiar with as the ‘bell curve’: thin tails surrounding a thicker middle, symmetrically centered around an average value. There’s a formula for the normal distribution, but for our purposes we don’t need to dwell too much on it. What’s important to understand is that the normal distribution is, essentially, just a type of shape with well understood properties, in the same way that ‘circles’ are a type of shape. Just like a circle can be larger or smaller, normal distributions come in different sizes — some are taller and narrower and some flatter and wider — but all have certain things in common. In order to perfectly describe a circle you only need two pieces of information, its radius and where its center is located. Similarly in order to perfectly describe a normal distribution you only need two pieces of information — its average value (analogous to its ‘center’) and its standard deviation.
Is a sample of 10 better than a sample of 1?
A sample of 10 is better than a sample of 1 and a sample of 100 is better than a sample of 10. This is the law of large numbers at work — the bigger your sample the closer to the true average you expect to be. You may realize that there are some caveats here, that it isn’t quite as simple as ‘more surveys equals better results’.
What is the law of large numbers?
The Law of Large Numbers states something that for many people may seem obvious: that the average value of numerous repeated trials should be about , well, the average expected value of any one of those trials. Any one trial might deviate from the average, but as you take more and more trials into consideration, their combined average will almost invariably move closer and closer to the expected average. A common example is with flipping a coin. If you flip enough times, you should get about half heads and half tails. If you were to only flip a coin a couple of times, it’s very possible to get a string of just heads or tails but as you keep going the probability of having a ratio of heads to tails that’s substantially different from the average drops away.
Is there a formula for normal distribution?
There’s a formula for the normal distribution, but for our purposes we don’t need to dwell too much on it. What’s important to understand is that the normal distribution is, essentially, just a type of shape with well understood properties, in the same way that ‘circles’ are a type of shape.
What is the central limit theorem?
Central limit theorem is a statistical theory which states that when the large sample size is having a finite variance, the samples will be normally distributed and the mean of samples will be approximately equal to the mean of the whole population.
What is the CLT in statistics?
The CLT can be applied to almost all types of probability distributions. But there are some exceptions. For example, if the population has a finite variance. Also this theorem applies to independent, identically distributed variables. It can also be used to answer the question of how big a sample you want. Remember that as the sample size grows, the standard deviation of the sample average falls because it is the population standard deviation divided by the square root of the sample size. This theorem is an important topic in statistics. In many real time applications, a certain random variable of interest is a sum of a large number of independent random variables. In these situations, we can use the CLT to justify using the normal distribution.
What is CLT used for?
5] CLT is used in calculating the mean family income in a particular country.
What is the probability that the weight of the cylinder is less than 28 kg?
Thus the probability that the weight of the cylinder is less than 28 kg is 38.28%. Example 3: The record of weights of female population follows normal distribution. Its mean and standard deviation are 65 kg and 14 kg respectively.
When is sample distribution assumed to be normal?
1] The sample distribution is assumed to be normal when the distribution is unknown or not normally distributed according to Central Limit Theorem. This method assumes that the given population is distributed normally. It helps in data analysis.
When to use t-score instead of z-score?
Since the sample size is smaller than 30, use t-score instead of the z-score, even though the population standard deviation is known.
What is the central limit theorem?
the Central Limit Theorem states that the sampling distribution of the mean of any distribution will be normal, provided that the sample size is large enough.
What is the CLT in statistics?
The CLT is foundational to hypothesis testing, the branch of inferential statistics that helps us draw conclusions about a population from only a representative subset of data.
Why can't we go out and collect data from the entire population?
In most useful, real-world projects, we can’t go out and collect data from the entire population due to time and resource constraints. However, CLT empowers us to confidently go out, collect data from a subset of the population, and then use statistics to draw conclusions about the population.
Is the country population distribution normal?
Clearly, the country population distribution is not normal. Even then, the sampling distribution is normal, with the mean very close to the population mean.
Does it matter what the population distribution is?
It doesn’t matter what the population (original) distribution is. If we sample, and the sample is large enough, the final distribution of the sample mean will be normally distributed. Further, the mean of this sampling distribution will be approximately equal to the population mean.
What happens if you repeat the same experiment 100 times?
If we repeat the same experiment, we will get a different mean. If we repeat the same experiment 100 times, we will get 100 different (samples) mean values.
Is CLT valid for Cauchy distribution?
The CLT theorem is only valid if the mean and variance of the distribution to be modeled are finite. Consequently, this theorem is not applicable in the case of Cauchy distribution. If you want to dig further, check this simulation walk-through of two examples, one where CLT is applicable, and one where it is not.
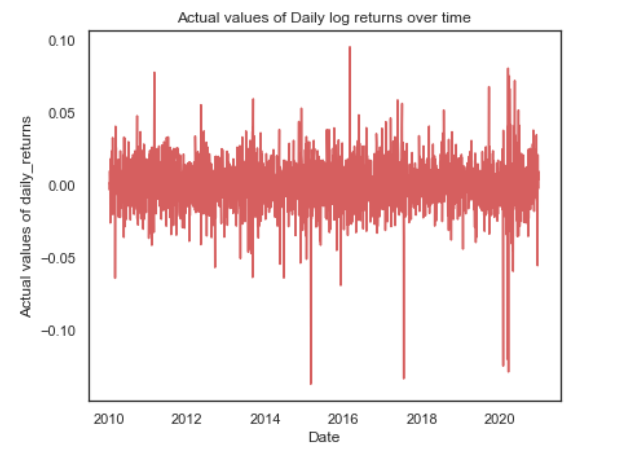
What Is The Central Limit Theorem?
Significance of Central Limit Theorem
Assumptions Behind The Central Limit Theorem
Why N ≥ 30 Samples?
Mean and Standard Deviation of The Sample
Implementation of Central Limit Theorem in Python
Conclusion
- The central limit theorem is a crucial concept in statistics and, by extension, data science. It's also crucial to learn about central tendency measures like mean, median, mode, and standard deviation. If you want to learn further, you can check the Data Scientist course by Simplilearn. The course gives exposure to key technologies including R, Pyt...
What Is The Central Limit Theorem (CLT)?
Understanding The Central Limit Theorem
The Central Limit Theorem in Finance
What Is The Central Limit Theorem
How Does The Central Limit Theorem Work
How Is Central Limit Theorem Applied in Data Science & Machine Learning
- One of the fundamental goals of any data analysistechnique is hypothesis testing. The basic question behind every hypothesis test is “does the data support my hypothesis or would I get the same data even if my idea was completely wrong?” In other words, you need to quantify the likelihood that you’re seeing the data due to chance and not due to you...
Central Limit Theorem Example