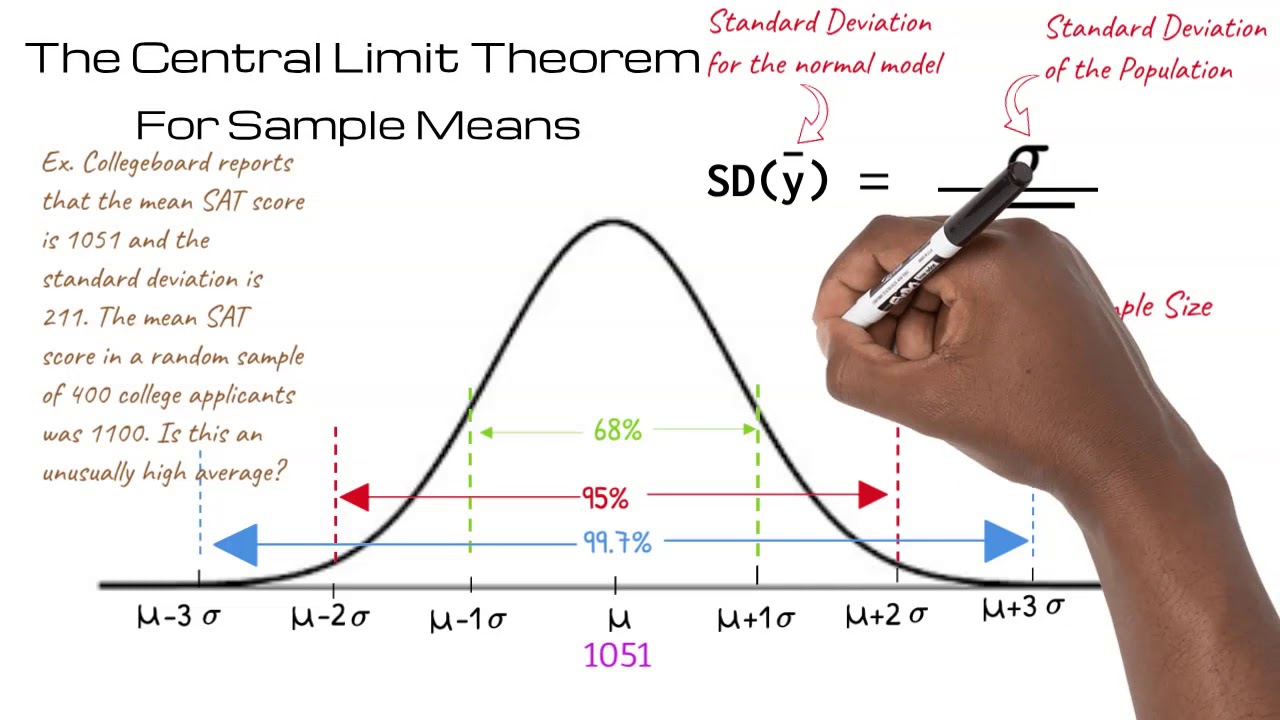
In order to apply the central limit theorem, there are four conditions that must be met:
- Randomization: The data must be sampled randomly such that every member in a population has an equal probability of being selected to be in the sample.
- Independence: The sample values must be independent of each other.
- The 10% Condition: When the sample is drawn without replacement, the sample size should be no larger than 10% of the population.
- Large Sample Condition: The sample size needs to be sufficiently large.
How do you calculate the central limit theorem?
μ = E ( X i) = r = 3. while the variance of a chi-square random variable with three degrees of freedom is: σ 2 = V a r ( X i) = 2 r = 2 ( 3) = 6. The Central Limit Theorem, therefore, tells us that the sample mean X ¯ is approximately normally distributed with mean: μ X ¯ = μ = 3. and variance: σ X ¯ 2 = σ 2 n = 6 n.
What does the central limit theorem enable researchers to do?
The central limit theorem tells us exactly what the shape of the distribution of means will be when we draw repeated samples from a given population. Specifically, as the sample sizes get larger, the distribution of means calculated from repeated sampling will approach normality.
What does the central limit theorem require?
when using the central limit theorem, if the original variable is not normal, a sample size of 30 or more is needed to use a normal distribution to the approximate the distribution of the sample means. The larger the sample, the better the approximation will be. The formula, z= x̄ -μ / (σ/√n) is used to gain information about a sample mean
What is so important about the central limit theorem?
- It can be used for making confidence intervals.
- It is able to disregard the distribution that some underlying X follows.
- The distribution of a sum approaches the normal distribution. This occurs while the distribution of terms in the underlying distribution are not necessarily normal.
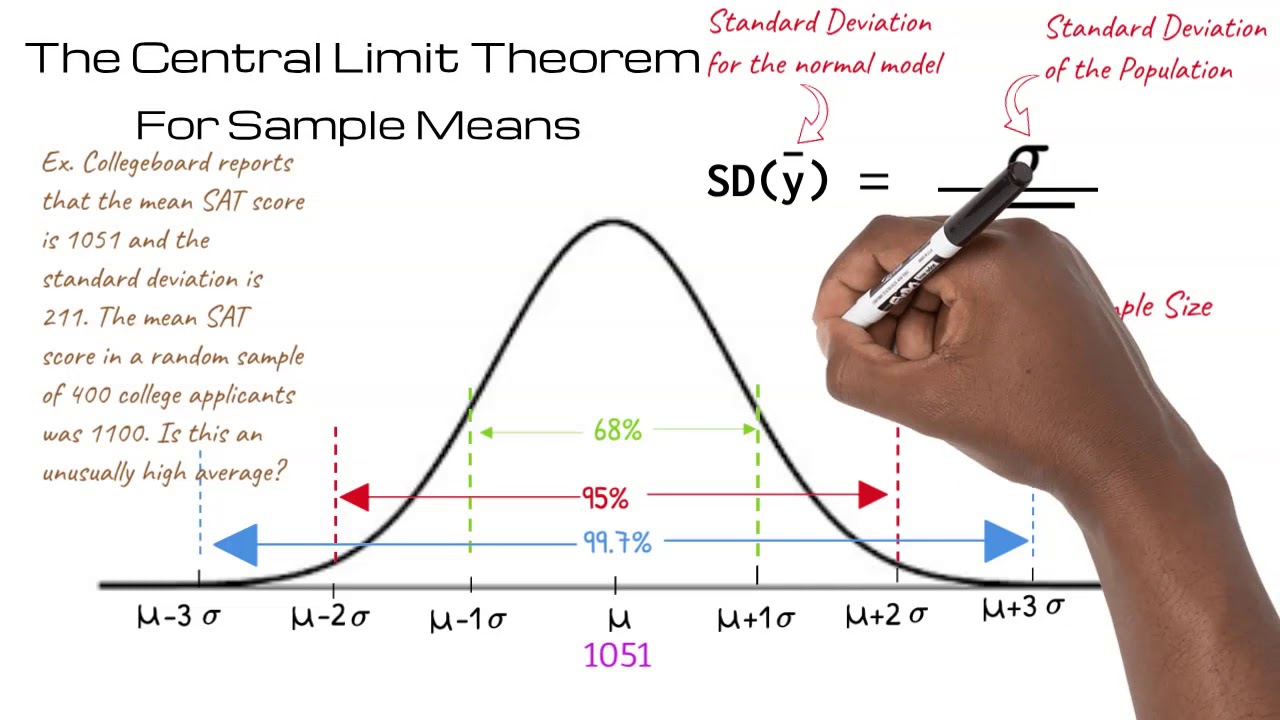
What are the conditions for central limit theorem to hold?
Key Takeaways. The central limit theorem (CLT) states that the distribution of sample means approximates a normal distribution as the sample size gets larger, regardless of the population's distribution. Sample sizes equal to or greater than 30 are often considered sufficient for the CLT to hold.
How do you verify the conditions of the central limit theorem?
0:014:08AP Statistics: Central Limit Theorem Conditions - YouTubeYouTubeStart of suggested clipEnd of suggested clipIf we were to sample sizes of some given value n. We would get an average. And if we repeated thatMoreIf we were to sample sizes of some given value n. We would get an average. And if we repeated that process over. And over again we would get lots of averages.
What are the conditions of the central limit theorem Why is central limit theorem important?
What is the Central Limit Theorem? The CLT is a statistical theory that states that - if you take a sufficiently large sample size from a population with a finite level of variance, the mean of all samples from that population will be roughly equal to the population mean.
What conditions are required by the central limit theorem quizlet?
What conditions are required by the central limit theorem before a confidence interval of the population mean may be created? The underlying population need not be normally distributed if the sample size is 30 or more.
Are the conditions for using the CLT fulfilled?
Yes, all the conditions for using the CLT are fulfilled. What would be the shape of the approximate sampling distribution of many means, each from a sample of 4 students? 4) The shape cannot be determined.
Is central limit theorem only for means?
This part of the definition refers to the distribution of the variable's values in the population from which you draw a random sample. The central limit theorem applies to almost all types of probability distributions, but there are exceptions.
What is the central limit theorem equation?
The central limit theorem gives a formula for the sample mean and the sample standard deviation when the population mean and standard deviation are known. This is given as follows: Sample mean = Population mean = μ μ Sample standard deviation = (Population standard deviation) / √n = σ / √n.
Which one of the following statements is true about central limit theorem?
C. The Central Limit Theorem states that the standard deviation of a sampling distribution of means (with N>1) will have a larger standard deviation than the population distribution from which it is taken.
What does the central limit theorem state Mcq?
What does the central limit theorem state? Explanation: The central limit theorem states that if the sample size increases sampling distribution must approach normal distribution.
What is the central limit theorem when does it apply quizlet?
Central Limit Theorem (CLT) tells us that for any population distribution, if we draw many samples of a large size, nn, then the distribution of sample means, called the sampling distribution, will: Be normally distributed.
What three things does the central limit theorem tell us?
To wrap up, there are three different components of the central limit theorem: Successive sampling from a population. Increasing sample size. Population distribution....Understanding the central limit theoremµ is the population mean.σ is the population standard deviation.n is the sample size.
Which of the following conditions must be met for applying the central limit theorem for estimating proportions in a population?
The population size must be at least 10 times bigger than the sample size. To apply the Central Limit Theorem for Sample Proportions when the sample is collected without replacement, the population size must be at least 10 times bigger than the sample size.
How do you find the probability using the central limit theorem?
AnswerSince the sample mean tends to target the population mean, we have μx=μ=34. ... The central limit theorem states that for large sample sizes (n), the sampling distribution will be approximately normal.The probability that the sample mean age is more than 30 is given by: P(Χ>30)=normalcdf(30,E99,34,1.5)=0.9962.More items...•
What conditions must be met for a sampling distribution to be normal?
Normal: The sampling distribution of p^p, with, hat, on top needs to be approximately normal — needs at least 10 expected successes and 10 expected failures. Independent: Individual observations need to be independent. If sampling without replacement, our sample size shouldn't be more than 10% of the population.
How do you find the sample mean using central limit theorem?
The central limit theorem states that for large sample sizes (n), the sampling distribution will be approximately normal. The probability that the sample mean age is more than 30 is given by P(Χ > 30) = normalcdf (30,E99,34,1.5) = 0.9962.
What is the sample size of the central limit theorem?
The sample size must be 30 or higher for the central limit theorem to hold. One of the most important components of the theorem is that the mean of the sample will be the mean of the entire population. If you calculate the mean of multiple samples of the population, add them up, and find their average, the result will be the estimate ...
Who coined the central limit theorem?
History of the Central Limit Theorem. The initial version of the central limit theorem was coined by Abraham De Moivre, a French-born mathematician. In an article published in 1733, De Moivre used the normal distribution to find the number of heads resulting from multiple tosses of a coin. The concept was unpopular at the time, ...
What is Bayes' theorem?
Bayes' Theorem The Bayes theorem (also known as the Bayes’ rule) is a mathematical formula used to determine the conditional probability of events. Central Tendency.
What is the law of large numbers?
Law of Large Numbers In statistics and probability theory, the law of large numbers is a theorem that describes the result of repeating the same experiment a large number of. Total Probability Rule.
Who invented the normal distribution?
However, in 1812, the concept was reintroduced by Pierre-Simon Laplace, another famous French mathematician. Laplace re-introduced the normal distribution concept in his work titled “Théorie Analytique des Probabilités,” where he attempted to approximate binomial distribution with the normal distribution.
What Is the Central Limit Theorem (CLT)?
In probability theory, the central limit theorem (CLT) states that the distribution of a sample variable approximates a normal distribution (i.e., a “bell curve”) as the sample size becomes larger, assuming that all samples are identical in size, and regardless of the population's actual distribution shape.
How many randomly selected stocks should be sampled for central limit theorem?
In this scenario, that investor may simply study a random sample of stocks to cultivate estimated returns of the total index. To be safe, use at least 30-50 randomly selected stocks across various sectors, should be sampled for the central limit theorem to hold. Furthermore, previously selected stocks must be swapped out with different names to help eliminate bias.
What does CLT mean?
The central limit theorem (CLT) states that the distribution of sample means approximates a normal distribution as the sample size gets larger. Sample sizes equal to or greater than 30 are considered sufficient for the CLT to hold.
What is the key aspect of CLT?
A key aspect of CLT is that the average of the sample means and standard deviations will equal the population mean and standard deviation. A sufficiently large sample size can predict the characteristics of a population accurately.
What is the size of a sample for a CLT?
As a general rule, sample sizes equal to or greater than 30 are deemed sufficient for the CLT to hold, meaning that the distribution of the sample means is fairly normally distributed. Therefore, the more samples one takes, the more the graphed results take the shape of a normal distribution.
Why is CLT important?
The CLT is useful when examining the returns of an individual stock or broader indices, because the analysis is simple, due to the relative ease of generating the necessary financial data. Consequently, investors of all types rely on the CLT to analyze stock returns, construct portfolios, and manage risk.
Is the mean of a sample of data closer to the mean of the overall population?
According to the central limit theorem, the mean of a sample of data will be closer to the mean of the overall population in question, as the sample size increases, notwithstanding the actual distribution of the data. In other words, the data is accurate whether the distribution is normal or aberrant. As a general rule, sample sizes equal to ...
What is the central limit theorem?
The central limit theorem states that the sampling distribution of a sample mean is approximately normal if the sample size is large enough, even if the population distribution is not normal.
How large of a sample is sufficient for the central limit theorem?
There is no exact definition for how large a sample size needs to be in order for the central limit theorem to apply, but in general it depends on the skewness of the population distribution that the sample comes from: If the population distribution is symmetric, sometimes a sample size as small as 15 is sufficient.
What does the central limit theorem state about sampling distribution?
Recall that the central limit theorem states that the sampling distribution of a sample mean is approximately normal if the sample size is “large enough”, even if the population distribution is not normal.
What is the central limit theorem?
In probability theory, the central limit theorem ( CLT) establishes that, in many situations, when independent random variables are added, their properly normalized sum tends toward a normal distribution (informally a bell curve) even if the original variables themselves are not normally distributed.
Who discovered the central limit theorem?
The central limit theorem has an interesting history. The first version of this theorem was postulated by the French-born mathematician Abraham de Moivre who, in a remarkable article published in 1733, used the normal distribution to approximate the distribution of the number of heads resulting from many tosses of a fair coin. This finding was far ahead of its time, and was nearly forgotten until the famous French mathematician Pierre-Simon Laplace rescued it from obscurity in his monumental work Théorie analytique des probabilités, which was published in 1812. Laplace expanded De Moivre's finding by approximating the binomial distribution with the normal distribution. But as with De Moivre, Laplace's finding received little attention in his own time. It was not until the nineteenth century was at an end that the importance of the central limit theorem was discerned, when, in 1901, Russian mathematician Aleksandr Lyapunov defined it in general terms and proved precisely how it worked mathematically. Nowadays, the central limit theorem is considered to be the unofficial sovereign of probability theory.
What is random orthogonal matrix?
A random orthogonal matrix is said to be distributed uniformly, if its distribution is the normalized Haar measure on the orthogonal group O (n,ℝ); see Rotation matrix#Uniform random rotation matrices .
What is a linear function of a matrix?
A linear function of a matrix M is a linear combination of its elements (with given coefficients), M ↦ tr (AM) where A is the matrix of the coefficients; see Trace (linear algebra)#Inner product .
What is asymptotic normality?
Asymptotic normality, that is, convergence to the normal distribution after appropriate shift and rescaling, is a phenomenon much more general than the classical framework treated above , namely, sums of independent random variables (or vectors). New frameworks are revealed from time to time; no single unifying framework is available for now.
What is log normal distribution?
Therefore, when the logarithm of a product of random variables that take only positive values approaches a normal distribution, the product itself approaches a log-normal distribution. Many physical quantities (especially mass or length, which are a matter of scale and cannot be negative) are the products of different random factors, so they follow a log-normal distribution. This multiplicative version of the central limit theorem is sometimes called Gibrat's law .
Is the characteristic function of a convolution the product of the characteristic functions of the densities involved?
Since the characteristic function of a convolution is the product of the characteristic functions of the densities involved, the central limit theorem has yet another restatement: the product of the characteristic functions of a number of density functions becomes close to the characteristic function of the normal density as the number of density functions increases without bound, under the conditions stated above. Specifically, an appropriate scaling factor needs to be applied to the argument of the characteristic function.
How to find the central limit theorem?
1. Check the Central limit theorem conditions for sample means: 1 The sample data must be independent. The data are randomly sampled from the population so they are independent. 2 The sample size must be sufficiently large. The sample size = 30 so it is large according to the CLT.
What is the sample size of a CLT?
The sample size (n) is at least 30 to apply the CLT to sample means and the sampling distribution of the sample mean will be nearly normal, even if the underlying population distribution of individual observations is not normally distributed.
How many standard deviations are there in a normal distribution?
For any normal distribution, 95% of the data are within 2 (or more accurately 1.96) standard deviations from the mean.
What is the x axis of a histogram?
The x-axis is the individual incomes and the histogram has a right-skewed distribution and not centered around the population mean which is plotted as a vertical black line.
What Is The Central Limit Theorem?
Central Limit Theorem Formula
- Fortunately, you don’t need to actually repeatedly sample a population to know the shape of the sampling distribution. The parametersof the sampling distribution of the mean are determined by the parameters of the population: 1. The meanof the sampling distribution is the mean of the population. 1. The standard deviationof the sampling distribution is the standard deviation of th…
Sample Size and The Central Limit Theorem
- The sample size (n) is the number of observations drawn from the population for each sample. The sample size is the same for all samples. The sample size affects the sampling distribution of the mean in two ways.
Conditions of The Central Limit Theorem
- The central limit theorem states that the sampling distribution of the mean will always follow a normal distributionunder the following conditions: 1. The sample size is sufficiently large. This condition is usually met if the sample size is n ≥ 30. 1. The samples are independent and identically distributed (i.i.d.) random variables. This condition...
Importance of The Central Limit Theorem
- The central limit theorem is one of the most fundamental statistical theorems. In fact, the “central” in “central limit theorem” refers to the importance of the theorem. The implication of the central limit theorem is that statistical theories that apply to normal distributions.
Central Limit Theorem Examples
- Applying the central limit theorem to real distributions may help you to better understand how it works.
What Is The Central Limit Theorem (CLT)?
Understanding The Central Limit Theorem
- According to the central limit theorem, the mean of a sample of data will be closer to the mean of the overall population in question, as the sample size increases, notwithstanding the actual distribution of the data. In other words, the data is accurate whether the distribution is normal or aberrant. As a general rule, sample sizes of around 30-50 are deemed sufficient for the CLT to h…
Key Components of The Central Limit Theorem
- The central limit theorem is comprised of several key characteristics. These characteristics largely revolve around samples, sample sizes, and the population of data. 1. Sampling is successive. This means some sample units are common with sample units selected on previous occasions. 2. Sampling is random. All samples must be selected at randomso th...
The Central Limit Theorem in Finance
- The CLT is useful when examining the returns of an individual stock or broader indices, because the analysis is simple, due to the relative ease of generating the necessary financial data. Consequently, investors of all types rely on the CLT to analyze stock returns, construct portfolios, and manage risk. Say, for example, an investor wishes to analyze the overall return for a stock in…