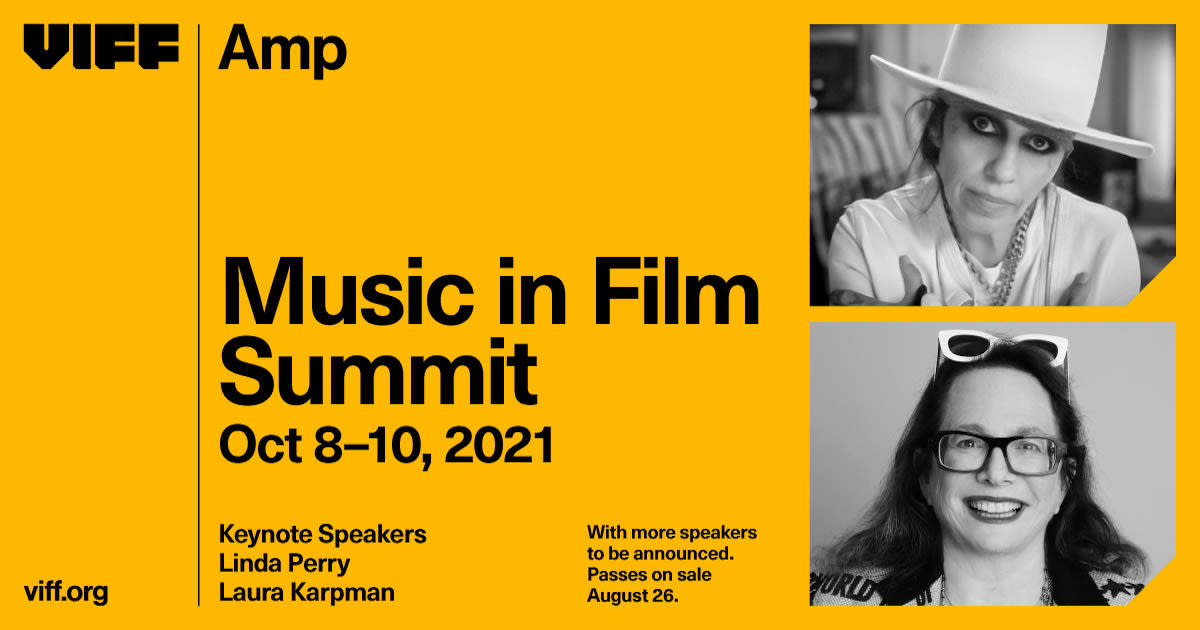
What is a high value of VIF?
In general, a VIF above 10 indicates high correlation and is cause for concern. Some authors suggest a more conservative level of 2.5 or above. Sometimes a high VIF is no cause for concern at all.
What is an acceptable VIF score?
Small VIF values, VIF < 3, indicate low correlation among variables under ideal conditions. The default VIF cutoff value is 5; only variables with a VIF less than 5 will be included in the model. However, note that many sources say that a VIF of less than 10 is acceptable.
What does VIF of 5 mean?
VIF > 5 is cause for concern and VIF > 10 indicates a serious collinearity problem.
What do you do when VIF is greater than 10?
A VIF value over 10 is a clear signal of multicollinearity. You also should to analyze the tolerance values to have a clear idea of the problem. Moreover, if you have multicollinearity problems, you could resolve it transforming the variables with Box Cox method.
What is considered high multicollinearity?
A rule of thumb to detect multicollinearity is that when the VIF is greater than 10, then there is a problem of multicollinearity.
What is acceptable VIF for multicollinearity?
Generally, a VIF above 4 or tolerance below 0.25 indicates that multicollinearity might exist, and further investigation is required. When VIF is higher than 10 or tolerance is lower than 0.1, there is significant multicollinearity that needs to be corrected.
What does VIF of 1 mean?
A VIF of 1 means that there is no correlation among the jth predictor and the remaining predictor variables, and hence the variance of bj is not inflated at all.
How do you deal with high VIF?
Try one of these:Remove highly correlated predictors from the model. If you have two or more factors with a high VIF, remove one from the model. ... Use Partial Least Squares Regression (PLS) or Principal Components Analysis, regression methods that cut the number of predictors to a smaller set of uncorrelated components.
How do you identify multicollinearity?
A simple method to detect multicollinearity in a model is by using something called the variance inflation factor or the VIF for each predicting variable.
Is multicollinearity really a problem?
Multicollinearity is a problem because it undermines the statistical significance of an independent variable. Other things being equal, the larger the standard error of a regression coefficient, the less likely it is that this coefficient will be statistically significant.
Is multicollinearity always a problem?
Depending on your goals, multicollinearity isn't always a problem. However, because of the difficulty in choosing the correct model when severe multicollinearity is present, it's always worth exploring.
What does VIF of 1 mean?
A VIF of 1 means that there is no correlation among the jth predictor and the remaining predictor variables, and hence the variance of bj is not inflated at all.
How do you interpret VIF values?
How to interpret the VIF. A VIF can be computed for each predictor in a predictive model. A value of 1 means that the predictor is not correlated with other variables. The higher the value, the greater the correlation of the variable with other variables.
What is VIF value in regression?
Variance inflation factor (VIF) is a measure of the amount of multicollinearity in a set of multiple regression variables. Mathematically, the VIF for a regression model variable is equal to the ratio of the overall model variance to the variance of a model that includes only that single independent variable.
What is VIF and tolerance?
Abstract. The variance inflation factor (VIF) and tolerance are two closely related statistics for diagnosing collinearity in multiple regression. They are based on the R-squared value obtained by regressing a predictor on all of the other predictors in the analysis. Tolerance is the reciprocal of VIF.
What does a VIF above 10 mean?
In general, a VIF above 10 indicates high correlation and is cause for concern. Some authors suggest a more conservative level of 2.5 or above. Sometimes a high VIF is no cause for concern at all. For example, you can get a high VIF by including products or powers from other variables in your regression, like x and x 2.
How to calculate a VIF?
VIFs are usually calculated by software, as part of regression analysis. You’ll see a VIF column as part of the output. VIFs are calculated by taking a predictor, and regressing it against every other predictor in the model. This gives you the R-squared values, which can then be plugged into the VIF formula. “i” is the predictor you’re looking at (e.g. x 1 or x 2 ):
What is the range of a VIF?
Therefore the range of VIF is between 1 and infinity.
How to calculate percentage of a VIF?
This percentage is calculated by subtracting 1 (the value of VIF if there were no collinearity) from the actual value of VIF:
What does infinite value mean in a VIF?
An infinite value of VIF for a given independent variable indicates that it can be perfectly predicted by other variables in the model.
When choosing a VIF threshold, should you take into account that multicollinearity is a lesser problem?
When choosing a VIF threshold, you should take into account that multicollinearity is a lesser problem when dealing with a large sample size compared to a smaller one. [ Source]
What is a VIF?
VIF measures the number of inflated variances caused by multicollinearity.
What does a VIF of 4 mean?
Generally, a VIF above 4 or tolerance below 0.25 indicates that multicollinearity might exist, and further investigation is required. When VIF is higher than 10 or tolerance is lower than 0.1, there is significant multicollinearity that needs to be corrected.
What is the reciprocal of a VIF?
The reciprocal of VIF is known as tolerance. Either VIF or tolerance can be used to detect multicollinearity, depending on personal preference. If R i2 is equal to 0, the variance of the remaining independent variables cannot be predicted from the i th independent variable. Therefore, when VIF or tolerance is equal to 1, ...
When high VIFs are caused as a result of the inclusion of the products or powers of other variables, does?
When high VIFs are caused as a result of the inclusion of the products or powers of other variables, multicollinearity does not cause negative impacts. For example, a regression model includes both x and x 2 as its independent variables. 3.
When a dummy variable represents more than two categories, does it have a high VIF?
When a dummy variable that represents more than two categories has a high VIF, multicollinearity does not necessarily exist . The variables will always have high VIFs if there is a small portion of cases in the category, regardless of whether the categorical variables are correlated to other variables.
Do high VIFs exist in control variables?
High VIFs only exist in control variables but not in variables of interest. In this case, the variables of interest are not collinear to each other or the control variables. The regression coefficients are not impacted. 2.
What does a VIF value mean?
A VIF can be computed for each predictor in a predictive model. A value of 1 means that the predictor is not correlated with other variables. The higher the value, the greater the correlation of the variable with other variables.
What is a VIF in deep learning?
The VIF can be applied to any type of predictive model (e.g., CART, or deep learning). A generalized version of the VIF, called the GVIF, exists for testing sets of predictor variables and generalized linear models.
What happens when the VIF is higher?
The higher the VIF, the more the standard error is inflated, and the larger the confidence interval and the smaller the chance that a coefficient is determined to be statistically significant.
What does a VIF of 1 mean?
From the above, we know that a VIF of 1 represents no multicollinearity, and higher values indicate more multicollinearity is present. What do these values actually mean?
What does the lowest possible VIF mean?
This is the lowest possible VIF and it indicates absolutely no multicollinearity. As R-squared increases, the denominator decreases, causing the VIFs to increase. In other words, as the set of IVs explains more of the variance in the individual IV, it indicates higher multicollinearity and the VIFs increase from 1.
How do you use VIFs in statistics?
Of course, the model has a dependent variable (Y), but we don’t need to worry about it for our purposes. When your statistical software calculates VIFs, it uses multiple regression to regress all IVs except one on that final IV. It repeats this process for all IVs, as shown below:
What is the VIF for independent variables?
The VIF for an independent variable equals the following: Where the subscript i indicates the independent variable. There is a VIF for each IV. When R-squared equals zero, there is no multicollinearity because the set of IVs does not explain any of the variability in the remaining IV.
Why Use VIFs Rather Than Pairwise Correlations?
Multicollinearity is correlation amongst the independent variables. Consequently, it seems logical to assess the pairwise correlation between all independent variables (IVs) in the model. That is one possible method. However, imagine a scenario where you have four IVs, and the pairwise correlations between each pair are not high, say around 0.6. No problem, right?
What is the limit value of a VIF?
Some papers argue that a VIF<10 is acceptable, but others says that the limit value is 5.
What is the smallest possible value for a VIF?
In chapter 3 of the book "An Introduction to Statistical Learning with Applications in R", it is said that "The smallest possible value for VIF is 1, which indicates the complete absence of collinearity. Typically in practice there is a small amount of collinearity among the predictors. As a rule of thumb, a VIF value that exceeds 5 or 10 indicates a problematic amount of collinearity" . This book was written by Gareth James , Daniela Witten , Trevor Hastie, and Robert Tibshirani.
Who wrote the VIF?
This book was written by Gareth James , Daniela Witten , Trevor Hastie , and Robert Tibshirani. A VIF is a useful starting point, but only a starting point. You can interpret its reciprocal, 1/VIF as 1 - R^2, where the R^2 comes from the regression of that predictor on the other predictors.
Transparency is our policy. Learn how it impacts everything we do
Transparency is how we protect the integrity of our work and keep empowering investors to achieve their goals and dreams. And we have unwavering standards for how we keep that integrity intact, from our research and data to our policies on content and your personal data.
How we make money
We sell different types of products and services to both investment professionals and individual investors. These products and services are usually sold through license agreements or subscriptions. Our investment management business generates asset-based fees, which are calculated as a percentage of assets under management.
How we use your personal data
How we use your information depends on the product and service that you use and your relationship with us. We may use it to:
How we approach editorial content
Maintaining independence and editorial freedom is essential to our mission of empowering investor success. We provide a platform for our authors to report on investments fairly, accurately, and from the investor’s point of view.
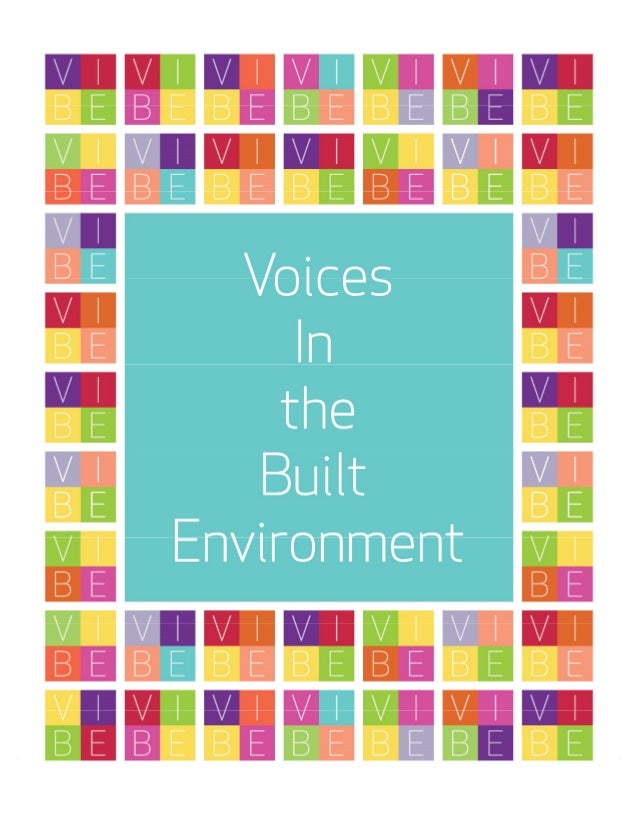
Variance Inflation Factor and Multicollinearity
Use of Variance Inflation Factor
Correction of Multicollinearity
More Resources